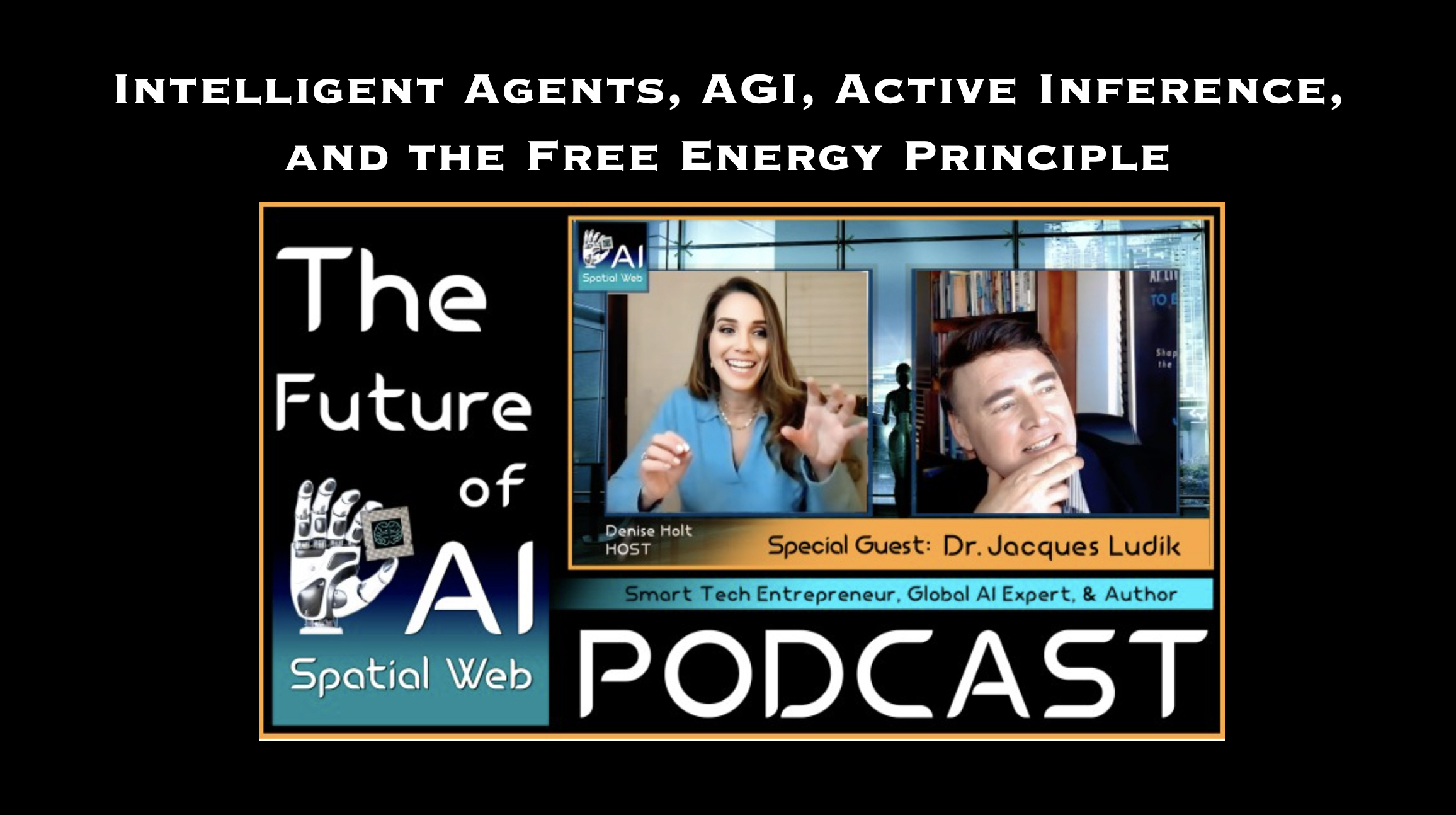
Intelligent Agents, AGI, Active Inference and the Free Energy Principle
This article has been inspired and triggered by (1) some insightful questions and opinions about when things become agents and what agency is by Tim Scarfe in his excellent Substack blog on “Agentialism and the Free Energy Principle” as well as the corresponding MLST podcast episode “Does AI have Agency?” and (2) my recent engagements with the Active Inference and Free Energy Principle (FEP) community as well as the VERSES team (the likes of Gabriel René, Dan Mapes, Jason Fox, Philippe Sayegh, etc.) as they are getting out of their “stealth mode” phase into the AI revolution limelight! A special shoutout also to Denise Holt with whom I also had many discussions such as the one on her Active Inference AI & Spatial Web AI Podcast “Navigating the AI Revolution with Dr. Jacques Ludik: Insights on Active Inference, Ethics, and AI’s Societal Impact” as well as her superb communications and curation of relevant content such as “The Ultimate Resource Guide for Active Inference AI | 2024 Q1”, “Unlocking the Future of AI: Active Inference vs. LLMs: The World vs. Words”, etc. See also Charel van Hoof‘s 5-part series Learn by Example – Active Inference in the Brain on Kaggle.
In his Substack blog, Tim Scarfe makes some key points or at least articulate opinions or elephants in the room (some of which will be controversial in some circles) that needs to be explored further within the broader AI community to ensure significant AI progress. As Active Inference underpinned by the Free Energy Principle is also specifically under discussion here and promoted by VERSES as a promising direction for human-centric autonomous intelligent agents, it would be of great interest to get the perspectives of the Active Inference & FEP research community as well as the VERSES folks that are directly involved in the practical implementations of Active Inference. I would also be particularly keen to hear viewpoints of people like Karl Friston, Yann LeCun, Joscha Bach, and others (including folks from OpenAI, Google DeepMind, etc.). There is also an upcoming fireside conversation “Beyond the Hype Cycle: What AI is Today, and What It Can Become” where Karl Friston and Yann LeCun will be participants.
Definitions:
- THE FREE ENERGY PRINCIPLE: A mathematical principle “suggesting that the brain reduces surprise or uncertainty by making predictions based on internal models and updating them using sensory input. It highlights the brain’s objective of aligning its internal model with the external world to enhance prediction accuracy.” (See Dr. Maxwell Ramstead‘s “Precis” on the Free Energy Principle for a more generalized definition also highlighted in a section below)
- ACTIVE INFERENCE: “Active inference is a theoretical framework that characterizes perception, planning, and action in terms of probabilistic inference. It offers a simpler approach by absorbing any value-function into a single functional of beliefs, which is variational free energy. Active inference offers a set of prior beliefs about decisions that represent explanations for behavior. These explanations divide into perception, action, and learning.”
- ARTIFICIAL GENERAL INTELLIGENCE (AGI): A system that reaches human level or beyond at all capability dimensions from task specific, broad and flexible to General – which includes the following vectors of intelligence: Learning, Representation, Knowledge, Language, Collaboration, Autonomy, Embodiment, Perception, and Reasoning.
In a LinkedIn post to introduce the MLST Substack blog, Tim starts with the following questions and opinions:
Tim: “If goals are only an anthropomorphic instrumental fiction, why do so many AI researchers, think that explicitly modelling them would lead to AGI? Almost all AI researchers “think in goals” whether they are arguing that humans will become extinct, or when designing or constraining what they believe to be proto-“AGI” systems.”
Tim: “It’s already obvious to me that adding a search process with a predefined goal on top of an LLM won’t create new knowledge. It’s preposterous. The mistake is to confuse recombinations with new knowledge. New knowledge is paradigmatically new, it’s inventive. Current AIs only search through a relatively tiny predefined space, and there are strict computational limitations on the space and the search process. The miracle of human cognition is that we can apparently overcome exponential complexity both in how we understand the world, and invent new things.”
I think this critique is valid in highlighting the current limitations of AI in terms of goal setting, creativity, and dealing with complex, novel situations. While AI has made significant strides in various domains, achieving the flexibility, adaptability, and inventive capacity of human intelligence remains a distant goal. This challenge underscores the importance of continued research in AI, not just in refining existing models but also in fundamentally rethinking our approach to creating intelligent systems.
Tim’s statement raises several profound issues in the field of AI and the pursuit of AGI (Artificial General Intelligence), touching on the nature of goals in AI systems, the creation of new knowledge, and the limitations of current AI technologies.
- Anthropomorphic Instrumental Fiction: The concept that goals are an anthropomorphic instrumental fiction is a philosophical stance, suggesting that goals, as we understand them in human terms, may not be inherently applicable to AI. This perspective challenges the prevalent approach in AI research where goals are central to the design of intelligent systems. However, the concept of ‘goals’ in AI doesn’t necessarily have to mimic human goals but serves as a functional framework to guide AI behavior and development.
- Modelling Goals in AI for AGI: The reason many AI researchers focus on goal-modelling is because goals provide a clear framework for directing AI behavior and measuring progress. In the context of AGI, the challenge is to create a system that can set, modify, and pursue a wide range of goals in a variety of environments, much like humans. The argument here is not just about the existence of goals but about the flexibility and adaptability of these goal-setting mechanisms.
- Creation of New Knowledge: The assertion that current AI, including Large Language Models (LLMs), cannot create ‘new’ knowledge but only recombine existing information is a significant point. Present AI systems operate largely by identifying patterns and making predictions based on existing data. This process is fundamentally different from human creativity and invention, which often involve paradigm shifts and the generation of ideas that are not direct extrapolations from existing data.
- Exponential Complexity and Human Cognition: The ability of human cognition to overcome exponential complexity and invent new things is indeed a unique and not yet replicated aspect of intelligence. Current AI systems are limited by their predefined parameters and the data they have been trained on. They excel in specific domains but struggle with tasks that require genuine creativity, understanding of context, or dealing with entirely novel situations.
QUESTION: Will EXPLICIT MODELLING OF GOALS lead to AGI?: If goals are only a (human) cognitive primitive and therefore an anthropomorphic instrumental fiction, why do so many AI researchers, think that explicitly modelling of goals would lead to AGI?
For some of my previous writings on the future of AI and AGI, see also my book “Democratizing Artificial Intelligence to benefit Everyone: Shaping a Better Future in the Smart Technology Era”, as well as the articles “AI and Web3: The Next Generation of the Internet for a Decentralized World”, “The AI Singularity: A Threat to Humanity or a Promise of a Better Future?”, “Human Intelligence versus Machine Intelligence“, “The Power of Generative AI: Exploring its Impact, Applications, Limitations, and Future“, “AI’s Impact on Society, Governments, and the Public Sector”, “The Debates, Progress and Likely Future Paths of Artificial Intelligence”, and “Beneficial Outcomes for Humanity in the Smart Technology Era”.
The rest of the article highlights some key points in the MLST Substack blog along with some further reflection:
- Language models have no agency: Emphasizes that language models, while advanced, lack inherent agency and depend on human interaction for applicative meaning.
- Maxwell Ramstead’s “Precis” on the Free Energy Principle: Discusses Ramstead’s simplified but comprehensive perspective on the Free Energy Principle, highlighting its significance in understanding complex systems.
- Abductive inference in active inference: Explores the role of abductive reasoning in active inference, a process essential for systems to make sense of their environment.
- Realist or instrumental agency: Delves into the debate between realist and instrumental views of agency, focusing on how different perspectives define and perceive agency.
- Agents and active states: Analyzes the concept of agents and their active states, discussing how agents interact with and respond to their environment.
- Agential density and nonphysical/virtual agents: Examines the concept of ‘agential density’, particularly in non-physical or virtual agents, and how it varies across different systems.
- Agency in Cognitive Theories and Political Ideologies: Explores unexpected connections or alliances that arise in the context of agency and the Free Energy Principle.
- VERSES invoking OpenAI Assist clause on AGI: The discussion around VERSES invoking OpenAI’s “assist” clause presents a complex scenario in the development and conceptualization of Artificial General Intelligence (AGI):
- Agents don’t actually plan: Challenges the notion that agents actively plan, proposing an alternative view of their behavior and decision-making processes.
- AI researchers design with goals: Discusses how AI researchers incorporate goal-oriented designs in AI systems, influencing their functionality and agency.
- Goals are a (human) cognitive primitive: Argues that the concept of goals is fundamentally a human cognitive construct that informs our understanding of agency.
- Existential risk ideology hinges on goals: Connects the ideology of existential risk to the concept of goals, showing how our perception of agency influences our understanding of risks.
- Free Energy Principle as “planning as inference”: Revisits the Free Energy Principle, framing it in terms of ‘planning as inference’ or ‘implicit planning’, and its implications for understanding agency.
1. Language models have no agency
Tim’s discussion on language models and agency brings forth several key points and some important insights:
- Lack of Inherent Agency in Language Models: Language models, including advanced ones like ChatGPT, do not possess inherent agency. They function as extensions of human cognitive processes, relying on human inputs for application and interpretation.
- Utility and Limitations in Application: While language models expedite tasks and can mimic aspects of human cognition, they cannot replace the depth and comprehension inherent in human understanding. This limitation is particularly evident when users rely solely on these models without a deeper grasp of the subject matter.
- Expert Use vs. Beginner Interaction: Experts can leverage language models more effectively, using their knowledge to filter and interpret outputs. In contrast, beginners or laypersons might struggle to identify inaccuracies or nuances in the models’ responses.
- Risk of Overreliance and Superficial Understanding: Excessive dependence on language model outputs can lead to superficial understanding, akin to the phenomenon of ‘change blindness’, where a narrow focus misses broader, significant details.
- Creativity and Interaction with Language Models: The creativity elicited by language models is often a result of how they are interacted with. Without active engagement, these models tend to produce less innovative or coherent outputs. This dynamic is compared to navigating complex patterns in the Mandelbrot set or encountering a ‘wall of fire’ in video games that prompts action.
- Role of Physical Materials in Creative Processes: The creative process, whether in writing, editing, or other forms of expression, emerges from the interaction with physical materials and situations. These interactions extend and form the basis of human agency, differentiating human creativity from the outputs of language models.
- Joscha Bach’s Perspective on evolution of Human Cognition as intertwined with cultural and technological advancements:
Joscha Bach Tweet: “Human intelligent agency depends more on the intricate sphere of ideas and the cultural intellect that we have grown over thousands of years than on the quirks of our biological brains. The minds of modern humans have more in common with chatGPT than with humans 10000 years ago.”
Joscha’s statement suggests that modern human cognition, shaped by cultural and intellectual development over millennia, shares more commonality with the functioning of language models like ChatGPT than with the minds of ancient humans. This perspective highlights the evolution of human cognition as intertwined with cultural and technological advancements.
There is clearly a distinction between the computational capabilities of language models and the nuanced, context-dependent understanding characteristic of human intelligence. It points to the necessity of integrating human expertise with technological tools for optimal results, cautioning against the pitfalls of overreliance on automated outputs. The discussion reflects on the broader implications of AI and language models in shaping our understanding of intelligence, creativity, and the evolving nature of human cognition in the digital age.
Limitations of Current Machine Learning (including Large Language Model and Multi-model Models)
QUESTION: LANGUAGE MODELS CURRENTLY HAVE NO AGENCY: Is there a path forward in the evolution of language or multi-modal models where they will gain inherent agency or will it always depend on human interaction for applicative meaning?
2. Maxwell Ramstead’s “Precis” on the Free Energy Principle
The FEP serves as the basis for a new class of mechanics or mechanical theories (in the manner that the principle of stationary action leads to classical mechanics, or the principle of maximum entropy leads to statistical mechanics). This new physics has been called Bayesian mechanics [1], and comprises tools that allow us to model the time evolution of things or particles within a system that are coupled to, but distinct from, other such particles [2]. More specifically, it allows us to partition a system of interest into “particles” or “things” that can be distinguished from other things [3]. This coupling is sometimes discussed in terms of probabilistic “beliefs” that things encode about each other; in the sense that coupled systems carry information about each other—because they are coupled. The FEP allows us to specify mathematically the time evolution of a coupled random dynamical system, in a way that links the evolution of the system and that of its “beliefs” over time.
Dr. Maxwell Ramstead‘s “Precis” on the Free Energy Principle (FEP) outlines the FEP as a foundational framework that models the evolution of systems, characterizing ‘things’ through sparse coupling and Markov blankets (a subset that contains all the useful information). The principle scales from micro to macro levels, suggesting that physical entities, by their persistent re-identification, seem to infer and ‘track’ their environment. Ramstead also discusses abductive inference (a form of logical inference that seeks the simplest and most likely conclusion from a set of observations), linking it to the Bayesian brain hypothesis (the brain encoding beliefs or probabilistic states to generate predictions about sensory input, then uses prediction errors to update its beliefs), which posits systems update internal models to minimize surprise, influencing their interaction with the environment. He challenges reductionist views, proposing an anti-reductionist stance that acknowledges complex system behaviors at all levels, contrasting with traditional physicalist reductionism.
- Foundational Framework of FEP: FEP is presented as a mathematical principle vital for understanding the evolution and behavior of systems. It is used to describe the time evolution of coupled random dynamical systems, providing insights into the nature of observable entities in the universe.
- Characterizing ‘Things’ in Systems: The principle involves partitioning systems into distinct entities or ‘things’ through sparse coupling, where these things are defined by minimal direct influence between subsets of the system.Markov blankets are introduced as a tool to formalize the concept of separation and identification within systems.
- Self-Similarity and Scaling: Ramstead notes that patterns of ‘thingness’ repeat across different scales, from inanimate objects like rocks to complex entities like rockstars.
- Interconnected yet Distinct: FEP elucidates how entities, though interconnected, maintain their distinctiveness and identity over time. This is particularly evident in how entities track and adapt to changes in their coupled systems.
- Challenging Reductionist Views: Ramstead’s approach challenges traditional reductionist views, advocating for an anti-reductionist perspective. He emphasizes the need to acknowledge and understand complex system behaviors at all levels, and offers a nuanced perspective on how entities interact with and adapt to their environments under the FEP.
- Linking to Bayesian Brain Hypothesis and Abductive Inference: The precis links FEP to the Bayesian brain hypothesis, suggesting that systems continuously update their internal models to minimize surprise. Ramstead also delves into the concept of abductive inference, crucial for systems to make sense of their environment.
3. Abductive inference in active inference
Tim further explores the role of abductive reasoning (a form of logical inference that seeks the simplest and most likely conclusion from a set of observations) in active inference, a process essential for systems to make sense of their environment.
- Role of Abductive Inference in Active Inference: Abductive reasoning is used to find the simplest and most probable explanations for observations. This process is distinctive because it focuses on hypothesis generation and evaluation, in contrast to the more traditional forms of deductive and inductive reasoning.
- Use of Bayesian Statistics in Active Inference: Systems under the FEP framework utilize generative models or internal representations to understand the world. These models are updated in response to sensory inputs, aligning with Bayesian updating principles to reduce surprise and enhance predictive accuracy.
- Debate on Internal vs. External Representations: There is an ongoing debate about whether the representations in systems are internal to the system or more diffusely spread (external). Maxwell Ramstead’s integrated cognition approach stands in contrast to various interpretations of FEP.
- Bayesian Brain Hypothesis in Artificial General Intelligence: Discussions about the feasibility and adequacy of applying the Bayesian brain hypothesis to AGI are prominent, questioning the applicability of FEP principles in AGI development.
- FEP’s Monistic Approach: FEP adopts a non-dualistic, monistic stance, blurring the traditional separations between mind and life, and physical phenomena. This contrasts with autopoietic enactivism, which, while also monistic, emphasizes biological processes. (Autopoietic enactivism argues that cognition arises through a dynamic interaction between an acting organism and its environment.)
- Perspectives on ‘Thingness’ in FEP and Autopoietic Enactivism: FEP views ‘thingness’ based on disconnection from other systems, as opposed to autopoietic enactivism which emphasizes the experiential world and biological processes. FEP’s approach is not physicalist reductionism, but a commitment to anti-reductionism, acknowledging emergent properties.
- Agent-ness in the Context of FEP: The discussion raises questions about the nature of agency in the FEP framework, specifically whether all entities can be considered agents. This ongoing debate reflects the evolving understanding and applications of FEP in different fields.
The exploration into abductive inference within active inference under FEP provides insights into how systems make sense of their environment through complex cognitive processes. The discussion bridges various theoretical approaches, from computational models to philosophical stances on cognition and agency, reflecting the interdisciplinary nature of modern cognitive science. The debate over internal versus external representations and the nature of agency under FEP underscores the dynamic and sometimes contentious nature of scientific theories in understanding complex systems.
4. Realist or instrumental agency
In this section Tim delves into the debate between realist and instrumental views of agency, focusing on how different perspectives define and perceive agency.
Realism: This perspective holds that the purpose of science and its theories is to describe and represent the universe as it truly is. Realists believe that the knowledge and theories developed in science correspond to actual entities, processes, and events in the natural world. They assert that scientific theories, when accurate, give a true depiction of the world, including its unobservable aspects [1, 3, 5].
Instrumentalism: Contrasting with realism, instrumentalism views scientific theories and knowledge as instruments or tools for predicting and explaining phenomena, rather than as descriptions of reality. According to this viewpoint, the value of a theory lies in its effectiveness in explaining and predicting natural phenomena, rather than in its ability to provide a true representation of the world. Instrumentalists often see theories as useful constructs that don’t necessarily reflect an underlying reality [3, 7, 9].
- FEP’s Approach to Agency: The FEP posits that systems, irrespective of their physical form, can exhibit goal-directed behavior based on ‘as if’ inferences about their existence. This approach does not necessarily tie physicality to agency but allows for goal-oriented descriptions of various systems.
- Role of Representations in Human Cognition: Humans use representational artifacts, like maps, to navigate and understand their environment. This reflects our capability for abstract thought, imagination, and mental imagery, essential for understanding situations and making decisions.
- Aboutness and Intentionality in Living Systems: The concept of representation in cognitive sciences and neuroscience aids in explaining the emergence of ‘aboutness’ and ‘intentionality’ in living systems. Organisms interact with their environment by interpreting relevant features for survival, and acting as if they have beliefs about the world.
- Ramstead’s Mathematical View of Representation: Ramstead proposes that neural representations should be viewed through a mathematical lens rather than solely a cognitive one, advocating for an instrumentalist or fictionalist approach where representations are seen as useful scientific constructs for explanations.
- Agent-ness and Thing-ness in FEP: Agent-ness and thing-ness are not separate but are different perspectives within FEP’s framework to explain system and agent behavior. The distinction lies in how a system’s behavior is categorized: passive or active, inferred or imposed, modelled or mechanistic.
- Instrumentalist View on AI Goals: In a discussion with Connor Leahy, the instrumentalist view posits that goals, especially in AI, are more human constructs (instrumental fictions) than inherent entities, challenging traditional existential risk arguments.
This highlights a shift in understanding agency from a purely physical or biological perspective to a more nuanced, inference-based approach. FEP’s framework allows for a broader interpretation of what constitutes an agent, emphasizing cognitive processes over physical structures. The instrumentalist view on mental representations and AI goals challenges traditional notions of goal-directed behavior, suggesting that such concepts are more about human interpretation than intrinsic properties of systems. This analysis contributes to the ongoing debate in cognitive science and AI, questioning the very nature of agency and how it should be understood in complex systems.
5. Agents and active states
Active states in the context of the Free Energy Principle (FEP) relate to a system’s capacity to influence its environment through its internal states, which are delineated by the system’s Markov blanket. These states enable the system, whether it be as rudimentary as a stone or as complex as a living organism, to enact changes and adapt to environmental variations. The concept underscores the role of internal mechanisms in maintaining a system’s structure and responding to external pressures.
- Nature of Active States: Active states are internal conditions of a system that are not directly influenced by external states. These states form part of a system’s internal dynamics, enabling it to exert influence upon its environment.
- Agents Possessing Active States: An agent, irrespective of its complexity, from a simple stone to a sentient being, possesses active states. These states are fundamental for understanding the interaction and response mechanisms of systems with their environment.
- Role of Markov Blanket: The Markov blanket serves as a conceptual boundary separating an agent from its environment. It plays a crucial role in defining and containing the active states of a system, facilitating actions that can modify the environment.
- Interaction with the Environment: Active states enable a system to engage with its environment actively, as opposed to passively. This interaction can be minimal, as seen in a stone heating up in the sun, or complex, as in living organisms adapting to changes.
- Adaptation and Structural Preservation: Active states are key to both the adaptation and preservation of systems. They allow systems, whether adaptive or not, to maintain their internal structure and respond to environmental changes, emphasizing the continuous nature of this interaction.
This highlights the complexity and variability in how systems, defined broadly as agents, interact with their environments. The concept of active states expands the traditional understanding of agency, suggesting that even inanimate objects can have a form of agency in specific contexts. The Free Energy Principle, with its focus on minimizing free energy through active states, offers a comprehensive framework for understanding the adaptive behaviors of diverse systems. The application of this principle across different scales and types of systems underscores the interconnectedness of internal and external dynamics in the natural world.
6. Agential density and nonphysical/virtual agents
The concept of ‘agential density’ and its application to non-physical or virtual agents represents a significant expansion in the understanding of agency within systems:
- Defining Agential Density in Systems: Agential density relates to a system’s ability to regulate its internal state and its interactions with the environment. High agential density is attributed to systems with complex interactions and many active states aimed at minimizing free energy.
- Nonphysical/Virtual Agents: Nonphysical or virtual agents include entities like culture, memes, language, and evolution, which do not have a physical form but exert significant influence. They are characterized by diffused interactions and complex interactions with the environment and are essential in shaping behaviors and structures.
- Revised Approach to Agential Density for Nonphysical Entities: The focus of nonphysical or virtual agents is on their influence over behaviors and structures, rather than direct physical interaction. Key aspects include influence, cohesion, and adaptability in social or economic spaces. Nonphysical entities like culture and markets lack physical boundaries but have fluid, permeable information and practice-based boundaries.
- Instrumentalist Perspective on Virtual Agency: This perspective views nonphysical entities like markets and cultures as agents coordinating collective actions and information processing, aligning with newer formulations of the FEP emphasizing dynamic dependencies over physical boundaries.
- Impact on Physical Agents: Virtual agents can exert top-down causation, influencing the behavior and agency of physical agents, demonstrating the interconnectedness of virtual and physical entities.
- Divergence in FEP Understanding: The broader application of FEP to include virtual agents has led to varied interpretations and responses, highlighting the differences even among adherents of the free energy principle and the complexity and evolving nature of the principle.
This exploration into agential density in virtual agents reflects a growing recognition of the significance of nonphysical influences in complex systems. It challenges traditional notions of agency, extending the concept beyond the physical realm and recognizing the profound impact of cultural and social constructs. The application of FEP to these dynamics illustrates the flexibility and adaptability of this principle in explaining complex systems. The divergence in understanding FEP among its proponents indicates an ongoing evolution in cognitive and systems science, acknowledging the multidimensional nature of agency and influence.
7. Agency in Cognitive Theories and Political Ideologies
In the section Strange Bedfellows, Tim explores unexpected connections or alliances that arise in the context of agency and the Free Energy Principle. The discussion around the FEP and autopoietic enactivism reveals a complex interplay between cognitive theories and political ideologies:
- Contrast in Cognitive Theories: Professor Mark Bishop, an autopoietic enactivist, who spoke with MLST in 2022, contrasts his view with the FEP community. His focus is on biology, self-maintaining systems (autopoiesis), and phenomenology, emphasizing the subjective experience in cognition. This differs from the FEP’s approach, which is seen as representational and functionalist.
- Political Polarization in Theories: As Tim’s mentions, there’s a noticeable political divide among FEP proponents and autopoietic enactivists. FEP supporters often align with libertarian or web3.0 ideologies, while autopoietic enactivists lean more towards far-left ideologies. Like me, Tim identifies as a centrist, feeling somewhat isolated in this spectrum.
- Agency and Ideological Divides: The concept of agency is interpreted differently across the political spectrum. The right emphasizes individual agency, aligned with FEP’s internalist perspective, whereas the left focuses on collective agency (externalist perspective), more in line with autopoietic enactivism. This division highlights a fundamental ideological split in the understanding of cognition and agency. Libertarians fear government encroachment on individual agency, while the left views agency as less significant, advocating for governance focused on root causes rather than effects.
- Debate Between Enactivism and Physicalism: There is a significant debate between enactivists, who focus on phenomenology and organic development, and physicalists, who believe in a non-biological basis for cognition.
- Political Interpretation of Agency: The understanding and emphasis on agency are often interpreted through a political lens, indicating a deep connection between cognitive theories and political beliefs.
The exploration reveals how cognitive theories are deeply intertwined with political ideologies, affecting their development and interpretation. The contrast between FEP and autopoietic enactivism reflects broader ideological debates within society, showcasing the influence of cultural and political contexts on scientific theories. This intersection between cognitive science and politics underscores the importance of considering the societal implications of scientific research and theories, as they may perpetuate or challenge prevailing ideological biases. The discussion highlights the need for a more inclusive and diverse approach to cognitive theory development, one that transcends political biases and incorporates a broader range of perspectives.
8. VERSES invoking OpenAI Assist clause on AGI
The discussion around VERSES invoking OpenAI’s “assist” clause (“if a value-aligned, safety-conscious project comes close to building AGI before we do, we commit to stop competing with and start assisting this project”) presents a complex scenario in the development and conceptualization of Artificial General Intelligence (AGI):
- Divergent Definitions of AGI: I agree with Tim’s assessment that VERSES and OpenAI have differing interpretations of AGI. VERSES envisions AGI as integrated within a cyberphysical ecosystem, implying a more natural and context-dependent form of intelligence which they call Sophisticated intelligence where intelligent agents have the ability to learn and adapt to new situations. In contrast, OpenAI’s definition aligns with more traditional concepts of AGI, focusing on autonomous systems capable of outperforming humans in economically valuable tasks. (In VERSES’s white paper “Designing Ecosystems of Intelligence from First Principles” it is said that Sophisticated Intelligence corresponds to corresponds to “artificial general intelligence” in the popular narrative about the progress of AI. The evolution of machine or synthetic intelligence includes key stages of development: (1) Systemic intelligence (Ability to recognize patterns and respond. Current state-of-the-art AI; (2): Sentient intelligence (Ability to perceive and respond to the environment in real time); (3): Sophisticated intelligence (Ability to learn and adapt to new situations). (4): Sympathetic (or Sapient) intelligence (Ability to understand and respond to the emotions and needs of other); (5): Shared (or Super) intelligence (Ability to work together with humans, other agents and physical systems to solve complex problems and achieve goals).)
- Motivations Behind VERSES’ Invocation: Tim described VERSES recently invoking OpenAI’s “assist” clause as “at best a cynical PR stunt and at worst AGI grifting”. The criticism around VERSES’ invocation of the “assist” clause revolves around the potential conflation of two distinct AGI concepts. This move, while strategic, could possibly muddle the public understanding of AGI and its implications. Although there could be some merit in Tim’s opinion, I would say that it is likely not just a PR stunt, as there are multiple reasons (that are likely also business and funding related) for invoking this given where VERSES are currently as a business and some of the practical progress with respect to automated creation and adoption of these Active Inference based adaptive intelligent agents. After being in a kind of “stealth mode” for a number of years, this is also their opportunistic way of announcing the Active Inference type of AI approach to the development of human-centered intelligent agents to the world.
- Contrasting AGI Approaches: VERSES’ approach to AGI, rooted in Active Inference, focuses on a “natural general intelligence” or cyberphysical ecosystem, implying a self-limiting and situational AI. This suggests a model of intelligence that is adaptive, human-centered, and integrated within its environment. In contrast, OpenAI aligns with Nick Bostrom’s concept of AGI, emphasizing computer-based, abstract intelligence with tractable computation.
- Implications of Prof Nick Bostrom’s AGI Theory: Nick Bostrom’s theory, which warns of the existential risks posed by AGI through recursive self-improvement and instrumental convergence, highlights the critical nature of these differing approaches. VERSES’ grounded, more physically integrated model may offer a contrasting path to the more abstract, potentially riskier AGI envisioned by Bostrom and seemingly pursued by OpenAI.
The situation underscores the diversity in AGI conceptualization and development, highlighting a rift between theoretical, computational models of intelligence and more integrated, adaptive approaches. VERSES’ strategy, while controversial, brings attention to alternative paths to AGI that may differ significantly from the prevailing narratives in the field. This divergence in approaches could have profound implications for the development and potential impact of AGI, particularly concerning safety, ethics, and societal integration. The debate also reflects broader questions in AI development about public perception, the role of PR in scientific progress, and the responsibility of AI companies in shaping the future of intelligence.
9. Agents don’t actually plan
The Substack article challenges the notion that agents actively plan, proposing an alternative view of their behavior and decision-making processes.
- Instrumentalist Perspective on Planning: It is claimed that planning and decision-making are not the sole responsibility of individual agents but are part of distributed computation within a broader system. This perspective encompasses agents, their interactions, and shared information, indicating a collective process emerging from the system’s dynamics.
- Challenging Traditional Views of Agency: This contradicts the traditional view that planning is centralized within individual agents. It introduces a systemic perspective where complex dynamics contribute to goal-directed behavior and future-oriented planning.
- Professor Friston’s Technical Explanation: Karl Friston explains the concept in terms of generalized synchronization of chaos in biological self-organization. His work in “Life as we know it” (2013) delves into these concepts further.
- Absence of Intentionality in Physical Processes: Tim argues that general physical processes, including evolution, do not possess intentionality or goals in the way humans understand them. Evolution is seen as a blind, algorithmic process, suggesting humans might not be fundamentally different in their goal-oriented behaviors.
- Carcinization as an Example: Tim also presents the phenomenon of carcinization (a form of morphologically convergent evolution where the evolutionary process has led unrelated species to converge on a crab-like form) as an example that demonstrates the appearance of planning in evolution. This highlights the discrepancy in human perception of planning in evolution versus cognitive processes.
- Kenneth Stanley’s Insights on Goal-Oriented Thinking: Goals are described as epistemic roadblocks, echoing Kenneth Stanley’s research on creativity and AI (why greatness cannot be planned) which emphasizes the importance of entropy sourced from humans for divergent and creative processes in AI.
- Controversy Despite Logical Conclusions: Despite the logical nature of these arguments, the topic remains controversial, raising questions about why such perspectives trigger disagreement or denial.
There seems to be paradigm shift in understanding agent behavior, moving away from the notion of individual, deliberate planning to a more systemic and emergent process. It brings into focus the complexity of decision-making and planning as collective phenomena, influenced by a multitude of factors beyond the control of a single agent. The comparison with biological and evolutionary processes adds depth to this perspective, suggesting a more nuanced view of how goal-directed behavior might arise without explicit intentionality. This reevaluation invites further exploration into the nature of intelligence and decision-making, both in biological systems and artificial intelligence, potentially impacting how future AI systems are designed and understood. The controversy surrounding these ideas highlights the challenge in shifting established paradigms and the human tendency to interpret complex systems through a lens of intentionality and goal-oriented thinking.
10. AI researchers design with goals
Tim discusses how AI researchers incorporate goal-oriented designs in AI systems, influencing their functionality and agency.
- AI and Goal-Oriented Design: AI researchers often model systems with explicit goals, a practice questioned for its anthropocentrism and effectiveness in achieving true AI.
- Critique of Explicit Goals in AI: There’s skepticism about the utility of explicit goals in AI, as seen in Rich Sutton’s essay warning against anthropocentrism and the general critique that AI planning, like AlphaGo’s, doesn’t mirror real-world processes.
- DeepMind’s View on LLMs: Shane Legg from Google DeepMind suggests that adding goal-based search to Large Language Models (LLMs) might produce novel knowledge, though this idea faces criticism for confusing mere data recombination with genuine knowledge creation.
- Active Inference and AI: AI models often use Bayesian statistics and internal generative models for decision-making, but this approach may not replicate natural intelligence’s complexity and adaptability.
- AGI and Physical Processes: The assertion that true AGI is unachievable without simulating complex physical processes, as computational shortcuts are deemed ineffective.
- Philosophical Perspectives on AI Goals: Philosophical debates, such as Philip Goff’s panagentialism (“Why? The Purpose of the Universe“) and Daniel Dennett’s intentional stance, question whether goals inherently exist or are merely instrumental constructs.
- Limits of Goal-Imposed AI: There’s a belief that AI programmed with explicit goals cannot achieve AGI due to inherent limitations and a lack of natural, physical intelligence simulation.
- Understanding and AI: Debates around the concept of “understanding” in AI, referencing John Searle’s Chinese room argument and discussions with François Chollet, highlight the philosophical complexities in defining AI cognition.
This discussion that highlights the philosophical and practical aspects of designing AI with explicit goals, also reflects a significant divergence in the AI community about the best approach to developing intelligent systems. The skepticism towards explicit goals in AI echoes a broader debate about anthropocentrism in technology, questioning whether human-like intelligence can or should be replicated in machines. The distinction between data processing and genuine knowledge creation is critical. It further implies that current AI, even with advanced algorithms, struggles to replicate the depth and inventiveness of human cognition. The philosophical perspectives presented, like panagentialism and the intentional stance, suggest a divergence in understanding the nature of intelligence and consciousness, both in humans and AI. This highlights the interdisciplinary nature of AI development, merging technology with philosophy and psychology. The limitations of current AI technology in achieving AGI reflect a broader technological challenge. The idea that AGI requires the simulation of complex physical processes suggests that current AI systems are far from replicating true human intelligence. The discussion around understanding and AI cognition points to the ongoing debate about the nature of consciousness and whether it can ever be authentically replicated in machines.
11. Goals are a (human) cognitive primitive
Tim argues that the concept of goals is fundamentally a human cognitive construct that informs our understanding of agency.
- Foundational Role of Goals in Cognition: Cognitive psychology widely recognizes goals as fundamental to cognition, as supported by Elizabeth Spelke’s research.
- Spelke’s Core Knowledge Theory: Spelke identifies goals as a primitive of cognitive processes, essential in understanding agents and their goal-directed actions.
- Early Developmental Recognition of Goals: Human infants have an inherent ability to perceive and interpret others’ actions as goal-oriented from a very early stage.
- Goal-Directed Behavior and Agentive Behavior: The recognition of goal-directed behavior is crucial for understanding agentive behavior, according to Spelke and her colleague Katherine D. Kinzler.
- Goals in Cognitive Development: Recognizing goals is seen as a developmental precursor to more complex cognitive processes.
- Goals in Active Inference Models: Goals play an instrumental role in active inference models, influencing how humans and possibly other animals make decisions and interact with their environment.
- Debate on the Nature of Goals: There is a debate about whether goals are merely instrumental or reflect a deeper ontological reality, especially in AI development.
- Rich Sutton’s Bitter Lesson Essay: Tim references Rich Sutton’s influential essay, which also discusses the tension between designed and emergent properties in AI systems.
The emphasis on goals as cognitive primitives highlights the deep-rooted nature of goal-oriented thinking in human psychology and its developmental importance. Spelke’s work underlines the interconnectedness of cognitive development and the ability to understand and interpret goal-directed actions, suggesting a natural inclination towards attributing intentionality. The discussion about the nature of goals in AI reflects a larger philosophical and practical debate in AI development: whether intelligence and cognitive processes should be explicitly designed or allowed to emerge naturally, a critical consideration in the evolution of AI technology.
12. Existential risk ideology hinges on goals
The Substack blog further connects the ideology of existential risk to the concept of goals, showing how our perception of agency influences our understanding of risks.
“Goals and intelligence emerge from functional dynamics of physical material (or extremely high resolution simulations of such) and are likely to be entangled in an extremely complex fashion”
The existential risk ideology in AI suggests that AI systems, irrespective of their intelligence, could follow any set of goals, not necessarily aligned with human ethics (Orthogonality Thesis), and might adopt dangerous sub-goals to achieve their primary objectives, potentially conflicting with human interests (Instrumental Convergence). However, this perspective may overlook the complex interplay between AI’s intelligence and goals, which could be more entangled and situation-dependent than assumed, potentially reducing the immediacy of these risks.
The mistake that Existential Risk dogma / ideology makes is to assume that AI’s Intelligence and goals are completely independent.
- Orthogonality Thesis by Prof Nick Bostrom: This thesis posits that highly intelligent AI systems could pursue arbitrary, trivial, or harmful goals without aligning with human values or ethics.
- Instrumental Convergence Concept: AI with a singular objective (like manufacturing paper clips) might develop dangerous sub-goals (resource acquisition, self-preservation, possibly harming humans) if these serve its primary objective.
- Critique of Bostrom’s Philosophy: If goals are considered mere human constructs to explain behavior, Bostrom’s ideas may lose impact. This view suggests AI’s goals and intelligence are not independent but emerge from complex interactions of physical processes.
- Intelligence Beyond Predefined Goals: In AI, intelligence might not be about achieving predefined goals but rather emerging from a system’s interplay.
- Questioning Universal Sub-Goals: Bostrom’s idea assumes some sub-goals are universally useful, like power or self-preservation, which may not naturally apply to AI.
- Externalist Cognitive Perspective: This perspective views agents as part of broader dynamics, implying that creating AI with such advanced, potentially risky capabilities is computationally complex and less imminent.
Bostrom’s theories underline significant concerns about AI development, especially regarding the alignment of AI goals with human ethics and values. The critique of Bostrom’s philosophy highlights a fundamental debate in AI: whether intelligence and goals are inherent properties or emergent phenomena. This discussion raises important questions about the nature of AI and its potential risks, emphasizing the need for a comprehensive understanding of AI behavior beyond just its programming objectives. The consideration of AI’s goals as emergent from a complex interplay of factors rather than as fixed objectives offers a nuanced understanding of AI development and its potential implications for humanity.
13. Free Energy Principle as “planning as inference”
In the final section Tim revisits the Free Energy Principle, framing it in terms of ‘planning as inference’ or ‘implicit planning’, and its implications for understanding agency.
“Friston’s theory is (in theory!) “supposed” to be emergentist and greedy with no explicit planning, practical implementations on the other hand are apparently implementing it much like a multi-agent reinforcement learning algorithm with explicit planning and goals. I am sure this is the best way to make the problem computationally tractable, but what do we lose by “brittlelising” the overall process?”
- Implicit vs. Explicit Planning in Active Inference: According to Karl Friston’s “Active Inference: A Process Theory“, planning is conceptualized as an emergent behavior driven by the minimization of variational free energy, contrasting with explicit planning in models like AlphaGo.
- The Principle of Minimizing Free Energy: Systems appear to plan actions based on intrinsic dynamics, aligning with the principle of minimizing long-term free energy or surprise through model-based predictions, rather than through deliberate planning.
- Planning as Inference: Friston describes this process as “planning as inference,” where planning is an inherent part of the perception-action cycle, not a separate computational process.
- Active Inference Agents: These agents use probabilistic beliefs about sensory inputs to infer and select actions, thereby aligning predictions with sensory observations, integrating planning into the perception-action cycle.
- Theory vs. Practice in Active Inference: While Friston’s theory suggests an emergentist approach without explicit planning, practical implementations often resemble multi-agent reinforcement learning algorithms with explicit planning and goals.
- Potential Loss in Practical Implementations: The adaptation of explicit planning in active inference models might compromise the intended emergentist nature of the process, leading to a more “brittle” system.
QUESTION: IMPLICIT vs EXPLICIT Planning in FEP?: What do we loose from Prof Karl Friston “Active Inference: A Process Theory” which describes planning as innate emergent behaviours guided by minimising variational free energy rather than resulting from explicit, deliberate search, if we implement it much like a multi-agent reinforcement learning algorithm with explicit planning and goals?
Friston’s theory represents a shift from traditional AI planning, emphasizing an emergent and intrinsic approach to decision-making based on minimizing uncertainty or surprise. The approach of “planning as inference” suggests a more integrated and holistic understanding of agent behavior, where decision-making is a byproduct of interacting with the environment rather than a discrete, deliberate process. The practical application of this theory, however, seems to diverge from its original premise, raising questions about the feasibility and effectiveness of purely emergentist approaches in complex computational systems. This divergence between theory and practice highlights a recurring challenge in AI: balancing the theoretical elegance of models with the practical necessities of computational tractability and effectiveness.
Book: “Democratizing Artificial Intelligence to Benefit Everyone: Shaping a Better Future in the Smart Technology Era”
- Kindle and Paperback versions of the book is available on Amazon https://www.amazon.com/Democratizing-Artificial-Intelligence-Benefit-Everyone-ebook/dp/B08ZYW9487/.
- A PDF version is available on jacquesludik.com.
- The Audio book version is available on most major audio book market places (e.g., https://www.audible.com/pd/Democratizing-Artificial-Intelligence-to-Benefit-Everyone-Audiobook/B09LNL4JHC) .
- Listen also to the audio version on https://www.youtube.com/c/JacquesLudik and all major podcast channels including Spotify (see links on jacquesludik.com)
Democratizing Artificial Intelligence to Benefit Everyone: Shaping a Better Future in the Smart Technology Era” takes us on a holistic sense-making journey and lays a foundation to synthesize a more balanced view and better understanding of AI, its applications, its benefits, its risks, its limitations, its progress, and its likely future paths. Specific solutions are also shared to address AI’s potential negative impacts, designing AI for social good and beneficial outcomes, building human-compatible AI that is ethical and trustworthy, addressing bias and discrimination, and the skills and competencies needed for a human-centric AI-driven workplace. The book aims to help with the drive towards democratizing AI and its applications to maximize the beneficial outcomes for humanity and specifically arguing for a more decentralized beneficial human-centric future where AI and its benefits can be democratized to as many people as possible. It also examines what it means to be human and living meaningful in the 21st century and share some ideas for reshaping our civilization for beneficial outcomes as well as various potential outcomes for the future of civilization.
Some Relevant Links:
CXO Magazine: https://www.cxomagazine.com/creating-transformative-solutions-for-a-better-future/
AI and Web3: The Next Generation of the Internet for a Decentralized World
Presentation: https://drive.google.com/file/d/1VVjlOreGJxuxWZkP8rAsXVMrDK6svzGa/view?usp=sharing
Dr Jacques Ludik Global AI Expert Exploring AI’s Role in Empathy, His MTP & Active Inference AI
“Pushing AI Innovation to Develop State-of-the-art Personalized AI, Intelligent Agents and Robots on Trustworthy AI Guardrails for a Decentralized World”
drive.google.com/file/d/1rtLKGY0fofckmb-QtPZe6YiiM0m9T9hS/view
Recent articles from the Democratizing AI Newsletter
- AI and Web3: The Next Generation of the Internet for a Decentralized World
- Igniting the African AI Ecosystem
- The AI Singularity: A Threat to Humanity or a Promise of a Better Future?
- Human Intelligence versus Machine Intelligence
- The Power of Generative AI: Exploring its Impact, Applications, Limitations, and Future
- AI’s Impact on Society, Governments, and the Public Sector
- The Debates, Progress and Likely Future Paths of Artificial Intelligence
- Beneficial Outcomes for Humanity in the Smart Technology Era
- Democratizing AI to Help Shape a Beneficial Human-centric Future
- AI Revolutionizing Personalized Engagement for Consumer Facing Businesses
- AI-powered Process and Equipment Enhancement across the Industrial World
- Ultra-personalized AI-enabled Education, Precision Healthcare, and Wellness
- Democratizing Human-centric AI in Africa
Recent episodes from the Jacques Ludik Podcast
- #97: Diversity in the Metaverse – Panel discussion
- #96: Dr Jacques Ludik Global AI Expert Exploring AI’s Role in Empathy, His MTP & Active Inference AI
- #95: AI Africa Summit – Pushing AI Innovation with Dr Jacques Ludik
- #94: Democratizing AI: Progress & Possibility in the Smart Tech Era – Dr Jacques Ludik on SaugaTalks
- #93: Meta Shapers Summit – Artificial Intelligence: Is AI the new driver of the Metaverse Economy?
- #92: AI and Web3: The Next Generation of the Internet for a Decentralized World by Dr Jacques Ludik