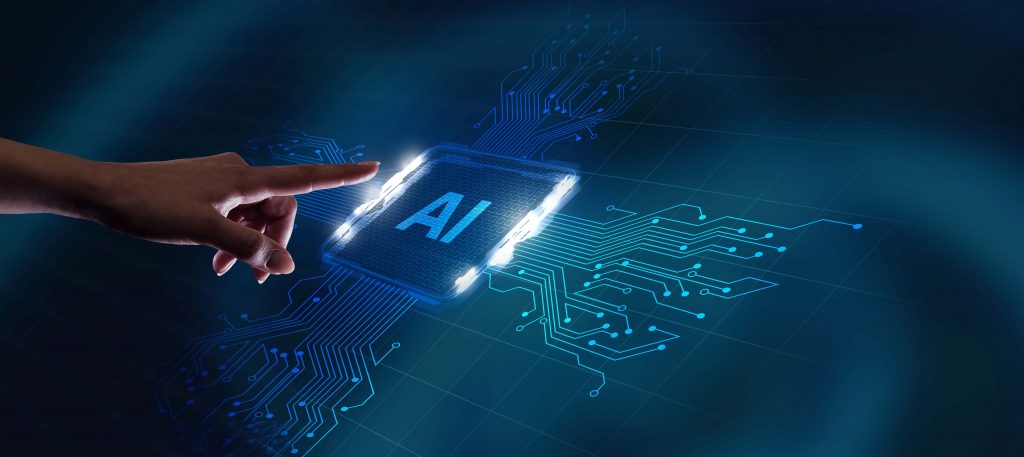
The Debates, Progress and Likely Future Paths of Artificial Intelligence
In this seventh article in the Democratizing AI series the focus is on the debates, progress and likely future paths of Artificial Intelligence. Over the last number of decades, we have seen AI’s impact, progress, and future direction being debated and discussed on so many different levels, AI research going through several rough “winter” and blossoming “summer” periods (actually all “seasons” of a year), as well as a variety of AI narratives, ideas, and perspectives of AI’s likely future paths. As we make scientific and engineering progress as humanity, we are steadily and surely getting better at building a rich and powerful toolbox of AI algorithms, structures, and techniques along with its software and hardware infrastructure for research and applications. We are also getting better at understanding the dynamics, power, nature, complexity and inner workings of AI and intelligence in a broader sense.
This article shares some text and audio extracts from Chapter 9, “The Debates, Progress and Likely Future Paths of Artificial Intelligence” in the book Democratizing Artificial Intelligence to Benefit Everyone: Shaping a Better Future in the Smart Technology Era. In this chapter, these aspects are explored in more detail to assist us in developing a more realistic, practical, and thoughtful understanding of AI’s progress and likely future paths that we can use as input to help shape a beneficial human-centric future. The following topics will also be discussed on 21 April 2022 at BiCstreet‘s “AI World Series” Live event (see more details at the bottom of the article):
- 32. Making Sense of the AI Debates
- 33. Human Intelligence versus Machine Intelligence
- 34. Lessons Learnt, Limitations, and Current State-of-the Art in AI
- 35. Progress, Priorities and Likely Future Paths for AI
(Previous articles in this series cover AI’s Impact on Society, Governments, and the Public Sector, Ultra-personalized AI-enabled Education, Precision Healthcare, and Wellness, “AI Revolutionizing Personalized Engagement for Consumer Facing Businesses“, “AI-powered Process and Equipment Enhancement across the Industrial World“, “AI-driven Digital Transformation of the Business Enterprise” as well as “AI as Key Exponential Technology in the Smart Technology Era” as further background.)
32. Making Sense of the AI Debates
The debates about AI’s future path and impact on humanity is like a roller-coaster ride of thoughts and ideas from so many different perspectives driven by a mix of fear and excitement about the enormous risks and opportunities that AI presents this century and beyond. As AI can be put firmly in the bracket of powerful enough technology that gives life “the potential to flourish like never before or to self-destruct” as described by the Future of Life Institute, the debates around AI and other smart technologies are becoming one of the most important discussions of our time.[i] Even with narrow AI systems that may accomplish a narrow set of goals at least as well as humans, these systems can in its own right or even within a connected network of such systems be powerful and impactful enough to create havoc or help humanity to thrive. In Nick Bostrom’s Superintelligence: Paths, Dangers, Strategies he reasons that strong AI in the form of intelligent machines that can match or outperform humans on any cognitive task and improve their capabilities at a faster rate than humans could potentially lead to an existential nightmare for humanity.[ii] He specifically believes that for such a strong AI to dominate, it would need to master skills such as intelligence amplification, strategizing, hacking, social manipulation, economic productivity, and technology research. Max Tegmark in Life 3.0, see strong AI as a third stage of life where technology designs its hardware (matter made of atoms) and software (information made of bits) as opposed to the first stage which is simple biological life that can only survive and replicate through evolving its hardware and software (information encoded in for example DNA) and the second stage which is more cultural where life can evolve its hardware (physical body) but also design its software such as humans learning new skills and knowledge and changing perspectives, goals and worldviews (Life 2.0).[iii] As today’s humans can perform minor hardware upgrades with for example implants, Max reckons that we are probably at a 2.1 level. According to the Future of Life Institute, most disputes amongst AI experts and others about strong AI that potentially have Life 3.0 capabilities, revolves around when and/or if ever it will happen and will it be beneficial for humanity.[iv] This leads to a classification where we have at least four distinct groups of thinking about where we are heading with AI which are the so-called Luddites, technological utopians, techno-skeptics, and the beneficial AI movement.[v] Whereas Luddites within this context are opposed to new technology such as AI and especially have very negative expectations of strong AI and its impact on society, technological utopians sit on the other end of the spectrum with very positive expectations of the impact of advanced technology and science to help create a better future for all. The Techno-sceptics do not think that strong AI is a real possibility within the next hundred years and that we should focus more on the shorter-term impacts, risks, and concerns of AI that can have a massive impact on society as also described in the previous chapter. The Beneficial-AI group of thinkers are more focused on creating safe and beneficial AI for both narrow and strong AI as we cannot be sure that strong AI will not be created this century and it is anyway needed for narrow AI applications as well. AI can become dangerous when it is developed to do something destructive or harmful but also when it is developed to do something good or advantageous but use a damaging method for achieving its objective. So even in the latter case, the real concern is strong AI’s competence in achieving its goals that might not be aligned with ours. Although my surname is Ludik, I am clearly not a Luddite, and would consider my own thinking and massive transformative purpose to be more aligned with the Beneficial AI group of thinkers and currently more concerned with the short-to-medium term risks and challenges and practical solutions to create a beneficial world for as many people as possible. Prominent business leaders, scientists, and influencers such as Elon Musk, the late Stephen Hawking, Martin Rees, and Eliezer Yudkowsky have issued dreadful warnings about AI being an existential risk to humanity, whilst well-resourced institutes countering this doomsday narrative with their own “AI for Good” or “Beneficial AI” narrative. AI researcher and entrepreneur Andrew Ng has once said that “fearing a rise of killer robots is like worrying about overpopulation on Mars”.[vi] That has also been countered by AI researcher Stuart Russell who said that a more suitable analogy would be “working on a plan to move the human race to Mars with no consideration for what we might breathe, drink, or eat once we arrive”.[vii] Many leading AI researchers seem to not identify with the existential alarmist view on AI, are more concerned about the short-to-medium term risks and challenges of AI discussed in the previous chapter, think that we are still at a very nascent stage of AI research and development, do not see a clear path to strong AI over the next few decades, and are of the opinion that the tangible impact of AI applications should be regulated, but not AI research and development. Most AI researchers and practitioners would fall into the beneficial AI movement and/or techno-sceptics category. Oren Etzioni, CEO of the Allen Institute for Artificial Intelligence, wrote an opinion article titled How to Regulate Artificial Intelligence where he claims that the alarmist view that AI is an “existential threat to humanity” confuses AI research and development with science fiction, but recognizes that there are valid concerns about AI applications with respect to areas such as lethal autonomous weapons, jobs, ethics and data privacy.[viii] From a regulatory perspective he proposes three rules that include that AI systems should be put through the full extent of the laws that apply to its human operator, must clearly reveal that they are not a human, and cannot keep or reveal confidential information without clear approval from the source of that information.
Some strong technological utopian proponents include roboticist Hans Moravec as communicated in his book Mind Children: The Future of Robot and Human Intelligence as well as Ray Kurzweil, who is currently Director of Engineering at Google and has written books on the technology singularity, futurism, and transhumanism such as The Age of Spiritual Machines and The Singularity is Near: When Humans Transcend Biology.[ix] The concept of a technological singularity has been popular in many science fiction books and movies over the years. Some of Ray’s predictions include that by 2029 AI will reach human-level intelligence and that by 2045 “the pace of change will be so astonishingly quick that we won’t be able to keep up, unless we enhance our own intelligence by merging with the intelligent machines we are creating”.[x] There are a number of authors, AI thought leaders and computer scientists that have criticized Kurzweil’s predictions in various degrees from both an aggressive timeline and real-world plausibility perspective. Some of these people include Andrew Ng, Rodney Brooks, Francois Chollet, Bruce Sterling, Neal Stephenson, David Gelernter, Daniel Dennett, Maciej Ceglowski, and the late Paul Allen. Web developer and entrepreneur Maciej Ceglowski calls superintelligence “the idea that eats smart people” and provides a range of arguments for this position in response to Kurzweil’s claims as well as Nick Bostrom’s book on Superintelligence and the positive reviews and recommendations that the book got from Elon Musk, Bill Gates and others.[xi] AI researcher and software engineer Francois Chollet wrote a blog on why the singularity is not coming as well as an article on the implausibility of an intelligence explosion. He specifically argues that a “hypothetical self-improving AI would see its own intelligence stagnate soon enough rather than explode” due to scientific progress being linear and not exponential as well as also getting exponentially harder and suffering diminishing returns even if we have an exponential growth in scientific resources. This has also been noted in the article Science is Getting Less Bang for its Buck that explores why great scientific discoveries are more difficult to make in established fields and notes that emergent levels of behavior and knowledge that lead to a proliferation of new fields with their own fundamental questions seems to be the avenue for science to continue as an endless frontier.[xii] Using a simple mathematical model that demonstrates an exponential decrease of discovery impact of each succeeding researcher in a given field, Francois Chollet concludes that scientific discovery is getting harder in a given field and linear progress is kept intact with exponential growth in scientific resources that is making up for the increased difficulty of doing breakthrough scientific research. He further constructs another model, with parameters for discovery impact and time to produce impact, which shows how the rate of progress of a self-improving AI converges exponentially to zero, unless it has access to exponentially increasing resources to manage a linear rate of progress. He reasons that paradigm shifts can be modeled in a similar way with the paradigm shift volume that snowballs over time and the actual impact of each shift decreasing exponentially which in turn results in only linear growth of shift impact given the escalating resources dedicated to both paradigm expansion and intra-paradigm discovery. Francois states that intelligence is just a meta-skill that defines the ability to gain new skills and should be along with hard work at the service of imagination, as imagination is the real superpower that allows one to work at the paradigm level of discovery.[xiii] The key conclusions that Francois makes in his article on implausibility of an intelligence explosion are firstly that general intelligence is a misnomer as intelligence is actually situational in the sense that the brain operates within a broader ecosystem consisting of a human body, an environment, and a broader society. Furthermore, the environment is putting constraints on individual intelligence which is limited by its context within the environment. Most of human intelligence is located in a broader self-improving civilization intellect where we live and that feeds our individual brains. The progress in science by a civilization intellect is an example of a recursively self-improving intelligence expansion system that is already experiencing a linear rate of progress for reasons mentioned above.[xiv]
In the essay The Seven Deadly Sins of Predicting the Future of AI, Rodney Brooks who is the co-founder of iRobot and Rethink Robotics, firstly quotes Amar’s law that “we tend to overestimate the effect of a technology in the short run and underestimate the effect in the long run” to state that the long term timing for AI is being crudely underestimated.[xv] He also quotes Arthur C. Clarke’s third law that states that “any sufficiently advanced technology is indistinguishable from magic” to make the point that arguments for a magical future AI are faith-based and when things said about AI that are far enough from what we use and understand today and for practical purposes passes the magic line, those things cannot be falsified. As it is also intuitive for us to generalize from the observed performance level on a particular task to competence in related areas, it is also natural and easy for us to apply the same human style generalizations to current AI systems that operate in extremely narrow application areas and overestimate their true competence level. Similarly, people can easily misinterpret suitcase words applied to AI systems to mean more than what there actually is. Rodney also argues that as exponentials are typically part of a S-curve where hyper growth flattens out, one should in general be careful to apply exponential arguments as it can easily collapse when a physical limit is hit or if there is not sufficient economic value to persist with it. The same holds for AI, where deep learning’s success, which can also be seen as an isolated event and achieved on top of at least thirty years of machine learning research and applications, does not necessarily guarantee similar breakthroughs on a regular basis. Not only is the future reality of AI likely to be significantly different to what is being portrayed in Hollywood science fiction movies, but also have a variety of advanced intelligent systems that evolve technologically over time in a world that would be adapting to these systems. The final error being made when predicting the future of AI is that the speed of deploying new ideas and applications in robotics and AI take longer than people think, especially when hardware is involved as with self-driving cars or in many factories around the world that are still running decades-old equipment along with old automation and operating system software.[xvi] On the self-driving cars front both Tesla and Google’s Waymo have improved self-driving technology significantly with Waymo achieving “feature complete” status in 2015 but in geo-fenced areas, whereas Tesla is at almost zero interventions between home and work (with an upcoming software release promising to be a “quantum leap”) in 2020.[xvii] However, the reality is that Tesla’s full driving Autopilot software is progressing much slower than what Elon Musk predicted over the years and Chris Urmson, the former leader of Google self-driving project and CEO of self-driving startup Aurora, reckons that driverless cars will be slowly integrated over the next 30 to 50 years.[xviii]
Piero Scaruffi, a freelance software consultant and writer, is even more of a techno-skeptic and wrote in Intelligence is not Artificial – Why the Singularity is not coming any time soon and other Meditations on the Post-Human Condition and the Future of Intelligence that his estimate for super intelligence that can be a “substitute for humans in virtually all cognitive tasks, including those requiring scientific creativity, common sense, and social skills” to be approximately 200,000 years which is the time scale of natural evolution to produce a new species that will be at least as intelligent as us.[xix] He does not think that we’ll get to strong AI systems with our current incremental approach and that the current brute-force AI approach is actually slowing down research in higher-level intelligence. He guesses that an AI breakthrough will likely have to do with real memory that have “recursive mechanisms for endlessly remodeling internal states”. Piero disagrees with Ray Kurzweil’s “Law of Accelerating Returns” and points out that the diagram titled “Exponential Growth in Computing” is like comparing the power of a windmill to the power of a horse and concluding that windmills will keep improving forever. There is also no differentiation between progress in hardware versus progress in software and algorithms. Even though there has been significant progress in computers in terms of its speed, size, and cost-effectiveness, that does not necessarily imply that we will get to human-level intelligence and then super intelligence by assembling millions of superfast GPUs. A diagram showing “Exponential Growth in Computational Math” would be more relevant and will show that there has been no significant improvement in the development of abstract algorithms that improve automatic learning techniques. He is much more impressed with the significant progress in genetics since the discovery of the double-helix structure of DNA in 1953 and is more optimistic that we will get to superhuman intelligence through synthetic biology.[xx]
A survey taken by the Future of Life Institute says we are going to get strong AI around 2050, whereas one conducted by SingularityNET and GoodAI at the 2018 Joint Multi-Conference on Human-Level AI shows that 37% of respondents believe human-like AI will be achieved within five to 10 years, 28% of respondents expected strong AI to emerge within the next two decades while only 2% didn’t believe humans will ever develop strong AI.[xxi] Ben Goertzel, SingularityNET’s CEO and developer of the software behind a social, humanoid robot called Sophia, said at the time that “it’s no secret that machines are advancing exponentially and will eventually surpass human intelligence” and also “as these survey results suggest, an increasing number of experts believe this ‘Singularity’ point may occur much sooner than is commonly thought… It could very well become a reality within the next decade.”[xxii] Lex Fridman, AI Researcher at MIT and YouTube Podcast Host thinks that we are already living through a singularity now and that super intelligence will arise from our human collective intelligence instead of strong AI systems.[xxiii] George Hotz, a programmer, hacker, and the founder of Comma.ai also thinks that we are in a singularity now if we consider the escalating bandwidth between people across the globe through highly interconnected networks with increasing speed of information flow.[xxiv] Jürgen Schmidhuber, AI Researcher and Scientific Director at the Swiss AI Lab IDSIA, is also very bullish about this and that we soon should have cost-effective devices with the raw computational power of the human brain and decades after this the computational power of 10 billion human brains together.[xxv] He also thinks that we already know how to implement curiosity and creativity in self-motivated AI systems that pursue their own goals at scale. According to Jürgen superintelligent AI systems would likely be more interested in exploring and transforming space and the universe than being restricted to Earth. AI Impacts has an AI Timeline Surveys web page that documents a number of surveys where the medium estimates for a 50% chance of human-level AI vary from 2056 to at least 2106 depending on the question framing and the different interpretations of human-level AI, whereas two others had medium estimates at the 2050s and 2085.[xxvi] Rodney Brooks has declared that artificial general intelligence has been “delayed” to 2099 as an average estimate in a May 2019 post that references a survey done by Martin Ford via his book Architects of Intelligence where he interviewed 23 of the leading researchers, practitioners and others involved in the AI field.[xxvii] It is not surprising to see Ray Kurzweil and Rodney Brooks at opposite ends of the timeline prediction, with Ray at 2029 and Rodney at 2200. Whereas Ray is a strong advocate of accelerating returns and believe that a hierarchical connectionist based approach that incorporates adequate real-world knowledge and multi-chain reasoning in language understanding might be enough to achieve strong AI, Rodney thinks that not everything is exponential and that we need a lot more breakthroughs and new algorithms (in addition to back propagation used in Deep Learning) to approximate anything close to what biological systems are doing especially given the fact that we cannot currently even replicate the learning capabilities, adaptability or the mechanics of insects. Rodney reckons that some of the major obstacles to overcome include dexterity, experiential memory, understanding the world from a day-to-day perspective, comprehending what goals are and what it means to make progress towards them. Ray’s opinion is that techno-sceptics are thinking linearly, suffering from engineer’s pessimism and do not see exponential progress in software advances and cross fertilization of ideas. He believes that we will see strong AI progresses exponentially in a soft take off in about 25 years.
(For more on this, read the paperback or e-book, or listen to the audiobook or podcast – see jacquesludik.com)
33. Human Intelligence versus Machine Intelligence
As we contemplate human intelligence versus machine intelligence, let us briefly consider here some broad definitions of intelligence. Those include defining intelligence to be “the ability to acquire and apply knowledge and skills” or “the ability to perceive or infer information, and to retain it as knowledge to be applied towards adaptive behaviors within an environment or context” or “the capacity for logic, understanding, self-awareness, learning, emotional knowledge, reasoning, planning, creativity, critical thinking, and problem-solving”.[i] Another summarized version in the context of AI research is that intelligence “measures an agent’s ability to achieve goals in a wide range of environments.”[ii] In considering the measure of intelligence Francois Chollet also emphasizes that both elements of achieving a task-specific skill as well as generality and adaptation as demonstrated through skill acquisition capability are key. He goes further and defines intelligence as a “measure of its skill-acquisition efficiency over a scope of tasks with respect to priors, experience, and generalization difficulty”.[iii] Following an Occam’s razor approach, Max Tegmark defines intelligence very broadly as the ability to accomplish complex goals, whereas Joscha Bach simply defines intelligence as the ability to make models, where a model is something that explains information, information is discernible differences at the systemic interface, and the meaning of information is the relationships that are discovered to changes in other information.[iv] Joscha’s definition for intelligence differs from Max’s one, as he sees achieving goals as being smart and choosing the right goals as being wise. So, one can be intelligent, but not smart or wise. For the “making models” definition of intelligence, aspects such as generality, adaptability, and skills acquisition capability with consideration of priors, experience, and generalization difficulty, would imply that the models being produced will be able to generalize and adapt to new information, situations, environments or tasks. The way an observer can find ground truth is by making models, and then build confidence in the models by testing it in order to determine if it is true and to which degree (which is also called epistemology). So, the confidence of one’s beliefs should equal the weight of the evidence. Language in its most general sense where it includes natural language and mental representation is the rules of representing and changing models. The set of all languages is what we call mathematics and is used to express and compare models. He defines three types of models: a primary model that is perceptual and optimizes for coherence, a knowledge model that repairs perception and optimizes for truth, and agents that self-regulate behavior programs and rewrite other models. He sees intelligence as a multi-generational property, where individuals can have more intelligence than generations, and civilizations have more intelligence than individuals.[v] For a human intellect, the mind is something that perceptually observes the universe, uses neurons and neurotransmitters as a substrate, have a working memory as the current binding state, a self that identifies with what we think we are and what we want to happen, and a consciousness that is the content of attention and makes knowledge available throughout the mind. A civilization intellect is similar but have a society that observes the universe, people and resources that function as the substrate, a generation that act as the current binding state, a culture (self of civilization) that identifies what we think we are and what we want to happen, and media (consciousness of civilization) that provides the contents of attention making knowledge available throughout society.[vi]
As Joscha Bach considers the mind of a human intellect to be a general modeling system with one or more paradigms that interface with the environment combined with universal motivation, he thinks we predominantly need better algorithms than what we currently have to make better generalized models. Our current solutions to modeling includes for example convex optimization using deep learning, probabilistic models, and genetic algorithms, whereas the general case of mental representation seems to be a probabilistic algorithm, which is hard to do with deep learning’s stochastic gradient descent based approach which is better at solving perceptual problems.[vii] If we look at how much source code we need to generate a brain, the Kolmogorov complexity (the length of a shortest software program that generates the object) as output is limited by a subset of the coding part of the genome involved in building a brain which is likely less than 1 Gigabyte (assuming a rough calculation where most of the 70 GB of the coding part of a genome’s 700 GB codes for what happens in a single cell and 1 GB codes for structural organization of the organism). If we assume from an implementation perspective that the functional unit in the human brain is likely cortical columns, the implementation complexity which can be calculated as the number of effective processing units and connectivity seems to be in the order of a few hundred Gigabytes.[viii] Given these high-level complexity calculations strong AI that emulates the human brain should in principle be possible. AI also has the ability to scale, whereas biological brains due to evolution run into limits such as the high level of metabolism that fast and large nervous systems need, the proportionally larger organisms that are required by large brains, longer training periods that are required for better performance, information flow being slowed down by splitting intelligence between brains, communication becoming more difficult with distance, and not having the interests between individuals fully aligned. AI systems on the other hand are more scalable with variable energy sources, reusable knowledge, cost-effective and reliable high bandwidth communication, as well as not having to align a multi-agent AI system or even have to make generation changes.[ix] Depending on the objectives and reward functions of AI systems and how it is aligned with human values as we want it to be, it does not need to be constrained by optimizing evolutionary fitness or adhering to physiological, social and cognitive constraints of biological systems, but can be more focused on achieving its goals which might in the broadest sense include optimizing its use of negative entropy. Scalable strong AI systems will likely only require consciousness when attention is needed to solve problems as the rest can be done on “autopilot” similar to how we do some activity that we have mastered well in automatic fashion without having to think about it.
Frank Wilczek, a Physics Professor at MIT, author of A Beautiful Question: Finding Nature’s Deep Design and recipient of the 2004 Nobel Prize in Physics, makes the “astonishing corollary” in The Unity of Intelligence, an essay in John Brockman’s Possible Minds: Twenty-five Ways of Looking at AI, that natural intelligence such as human intelligence is a special case of artificial intelligence.[x] He infers this by combining evidence of physics about matter with Francis Crick’s “astonishing hypothesis” in neurobiology that the mind emerges from matter, which is also the foundation for modern neuroscience. So, Frank claims that the human mind emerges from physical processes that we can understand and in principle reproduce in an artificial manner. For this corollary to fail he argues that some new significant phenomenon needs to be discovered that has “large-scale physical consequences, that takes place in unremarkable, well-studied physical circumstances (i.e., the materials, temperatures, and pressures inside human brains), yet has somehow managed for many decades to elude determined investigators armed with sophisticated instruments. Such a discovery would be … astonishing.”[xi] With respect to the future of intelligence, Frank concludes that the superiority of artificial over natural intelligence looks to be permanent, whereas the current significant edge of natural intelligence over artificial intelligence seems to be temporary. In support of this statement, he identifies a number of factors whereby information-processing smart technology can exceed human capabilities which includes electronic processing that is approximately a billion times faster than the latency of a neuron’s action potential, artificial processing units that can be up to ten thousand times smaller than a typical neuron which allows for more efficient communication, and artificial memories that is typically digital which enables it to be stored and maintained with perfect accuracy in comparison to human memory that is analog and can fade away. Other factors include human brains getting tired with effort and degrading over time, the artificial information processors having a more modular and open architecture to integrate with new sensors and devices compared to the brain’s more closed and non-transparent architecture, and quantum computing that can enable qualitatively new forms of information processing and levels of intelligence compared to seemingly not being suitable for interfacing with the human brain.[xii] That said, there are also a number of factors that give the human brain with its general-purpose intelligence an edge above current AI systems. These include the human brain making much better use of all three dimensions compared to the 2-dimensional lithography of computer boards and chips, the ability of the human brain to repair itself or adapt to damage whereas computers must typically be rebooted or fixed, and the human brain’s tight integration with a variety of sensory organs an actuators that makes interpreting internal and external signals from the real-world and the control of actuators seamless, automatic and with limited conscious attention. In addition to this, Frank conjectures that the two most far-reaching and synergistic advantages of human brains are their connectivity and interactive development, where neurons in the human brain typically have hundreds to thousands of meaningful connections as opposed to a few fixed and structured connections between processing units within computer systems, and the self-assembly and interactive shaping of the structure of the human brain through rich interaction and feedback from the real-world that we do not see in computer systems.[xiii] Frank Wilczek reckons that with humanity’s engineering and scientific efforts staying vibrant and not being derailed by self-terminating activities, wars, plagues or external non-anthropogenic events, we are on a path to be augmented and empowered by smart systems and see a proliferation of more autonomous AI systems that are growing in capability and intelligence.
As we get better at understanding ourselves and how our brains work, the more intelligent, precise, intuitive, and insightful our AI systems will become. Living in the age of knowledge, we are making advancements and discoveries at record paces.[xiv] Many researchers feel that we will not be able to create fully intelligent machines until we understand how the human brain works. In particular, the neocortex which is the six-layered mammalian cerebral cortex that is associated with intelligence and involved in higher-order brain functions such as cognition, sensory perception, spatial reasoning, language, and the generation of motor commands, needs to be understood before we can create intelligent machines.[xv] Not all people believe this. After all, aeroplanes fly without emulating birds. We can find ways to achieve the same goals without developing them to be perfect models of each other. For now, we are focusing on the brain and what people are doing to create AI systems that rely on our understanding of the brain. Neuroscience, in the last decade or two, perhaps has achieved massive success and a boost in the knowledge we have on how the brain works. In this, there are of course many theories and many different ways to look at it. Based on these different theories, there are different approaches to AI that can be created. The near future of machine intelligence is based on all of these coming together, and our current and past machine learning is based on parts of it, in a more hierarchical and networked manner. This means that as we understand more about the brain, we are enabled to create machines that are modeled on this working. The machines are then enabled to follow the brain’s process in its deductions, calculations and inferences of tasks and of reality in general. Perhaps what many people would like to know is if a machine can act like a human in its thinking and rational decision making, then how is it different from a human? Also, where does emotions fit in?
Lisa Feldman Barrett, a Professor of Psychology and Neuroscience at Northeastern University and author of books such as Seven and a Half Lessons About the Brain and How Emotions Are Made, have busted some neuroscience mythsof which one is the triune brain story that the brain evolved in layers consisting of the reptile brain (survival instincts), the limbic system (emotions) and the neocortex (rational thought and decision making), where the latter via the prefrontal cortex apply rational thought to control the survival instincts, animalistic urges and emotions.[xvi] Instead, as we contemplate the workings of the human brain in producing intelligence, it is important to keep in mind that our brain did not evolve so that we can think rationally, feel emotions, be imaginative, be creative or show empathy, but to control our bodies by predicting the body’s energy needs in advance in order to efficiently move, survive, and pass our genes to the next generation. It turns out the brains of most vertebrates develop in the same sequence of steps and are made from the same types of neurons but develop for shorter or longer durations which lead to species having different arrangements and numbers of neurons in their brains. Lisa’s theory of constructed emotion explains experience and perception of emotion in terms of multiple brain networks collaborating working together and what we see in the brain and body is only affect (i.e., the underlying experience of feeling, emotion, and mood).[xvii] This theory suggests that these emotions are concepts that are constructed by the brain and not biologically hardwired or produced in specific brain circuits. Instead, emotions are also predictions that emerge momentarily as the brain is predicting the feelings that one is expecting to experience. In fact, the brain is constantly building and updating predicting models of every experience we have or think we have. The brain guesses what might happen next and then prepares the body ahead of time to handle it. As all our sensory experiences of the physical world are simulations that happen so quickly that it feels like reactions, most of what we perceive is based on internal predictions with incoming external data simply influencing our perceptions. Another one of Lisa’s lessons is that we have the kind of nature that requires nurture as can be seen with the brains of babies and young children that wire themselves to their world and feed off physical and social inputs. As many brain regions that process language also controls the organs and systems in your body, we are not only impacting our own brain and body with our words and actions, but also influence the brains and bodies of other people around us in a similar way. Our brains are also very adaptable and create a large variation of minds or human natures that wires itself to specific cultures, circumstances, or social and physical environments. The final lesson is that our brains can create reality and are so good with believing our own abstract concepts and inventions, that we easily mistake social or political reality for the natural world. Lisa is also correct that not only do we have more control of the reality that we create, but we also have more responsibility for the reality than we think we have.[xviii] These types of insights into the human brain not only provide important context for understanding human intelligence, but also help us to think more clearly about the machine intelligence systems that we want to build to better support us.
In a talk about Planetary Intelligence: Humanity’s Future in the Age of AI, Joscha Bach gives a high-level layman’s rendition of our information processing cells which form a nervous system that regulates, learns, generalizes, and interprets data from the physical world.[xix] The nervous system has many feedback loops that take care of the regulation and whenever the regulation is insufficient the brain reinforces certain regulations via a limbic system that learns via pleasure and pain signals. Pleasure tells the brain to do more of what it is currently doing whereas pain tells it to do less. This is already a very good learning paradigm but has the drawback that it does not generalize very well. So, it needs to generalize across different types of pleasures and pains as well as predict these signals in the future. The next step is to have a system or an engine of motivation that implements the regulation of needs which can be physiological, social, and cognitive in nature. The hippocampus is a system that can associate needs, pleasure, and pain to situations in its environment, whereas the neocortex generalizes over these associations that are related to our needs. As it simulates a dynamic world, it determines what situations create pain or pleasure in different dimensions and what they have in common or not. A good metaphor is a synthesizer. Sound, for example, is a pattern generated by a synthesizer in your brain that makes it possible to predict patterns in the environment. So sound is being played by a synthesizer in your brain to make sense of the data in the physical world. Sound is just a particular class of synthesizers. Synthesizers do not only work for auditory patterns, but also for other modalities such as colors, spatial frequencies, and tactile maps. So, the brain can tune into the low-level patterns that it can see and then look for patterns within the patterns, which is called meta patterns. These meta patterns can then be linked together and that allows the brain to organize lots of different sounds into a single sound that for example only differs by pitch. So now there is a meta pattern that explains more of the sounds. The same holds for color, spatial frequencies, and other modalities. By lumping colors and spatial frequencies together visual percepts are obtained. At some point the neocortex merges the modalities and figures out that these visual patterns and sound patterns can be explained by mapping them to regions in the same 3-dimensional space. The brain can explain the patterns in the 3-dimensional space by assuming they are objects in the 3-dimensional space which are the same in different situations that are being experienced. To make that inference, the brain needs to have a conceptual representation in the address space of these mental representations that allow it to create possible words to generalize over the concepts that have been seen. In order to make that happen there are several types of learning involved such as function approximation that might include Bayesian, parallelizable or exhaustive modeling or scripts and schemas that might include sparse Markov Decision Processes, individual strategies and algorithms. The neocortex organizes itself into small circuits or basic units called the cortical columns that need to bind together into building blocks similar to Lego blocks to form representations for different contexts. The cortical columns form little maps that are organized into cortical areas and these maps interact with one another. These cortical areas play together like an orchestra, where a stream of music is being produced and every musician listens to the music around it and uses some of the elements to make their own music and pass it on. There is also a conductor that resolves conflicts and decides what is being played. Another metaphor is an investment bank, where there are lots of these cortical columns in the neocortex that are there to anticipate a reward for making models about the universe and of their own actions. The reward is given by management via a motivational system that effectively organizes itself into more and more hierarchies. It effectively functions like an AI built by an organism that learns to make sense of its relationship with the universe. The brain generates a model that produces a 3-dimensional world of hierarchies of synthesizers in the mind. As the brain is not a person but a physical system, it cannot feel anything – neurons cannot feel anything. So, in order to know what it’s like to feel something the brain finds it useful to create a story of a person that is playing out in the universe. This person is like a non-playing character being directed by the result of the brain’s activity and computed regulation. As the brain tells the story of the person as a simulated system, the story gets access to the language centre of the brain which allows it to express feelings and describes what it sees. Joscha believes that as consciousness is a simulated property, a physical system cannot be conscious and only a simulated system can be conscious.[xx] It is clear that the neocortex plays a key role in making models that learns, predicts, generalizes and interprets data from the physical world.
Jeff Hawkins, the co-founder and CEO of Numenta that aims to reverse-engineer the neocortex and enable machine intelligence technology based on brain theory, along with many researchers reckon that studying the human brain and in particular the neocortex is the fastest way to get to human-level AI.[xxi] As it stands now, none of the AI being developed is intelligent in the ways humans are. The initial theory Jeff and his team at Numenta has proposed is called Hierarchical Temporal Memory (HTM) which takes what it knows about the neocortex to build machine learning algorithms that are well suited for prediction, anomaly detection and sensorimotor applications, are robust to noise, and can learn time-based patterns in unlabeled data in continuous fashion as well as multiple patterns at the same time.[xxii] According to Jeff, the neocortex is no longer a big mystery and provides a high-level interpretation as follows: the human brain is divided into an old and new part (although Lisa Feldman Barret would say the “new part” is due to a longer development run). Only mammals have the new part which is the neocortex that occupies approximately 75% of the volume of the brain, whereas the old parts address aspects such as emotions, basic behaviors, and instincts. The neocortex is uniform – it looks the same everywhere – like it replicated the same thing over and over again, not divided into different parts that do separate things. The neocortex is like a very complex circuit that almost randomly sends signals to certain parts of the body. It seems very random. What we need is to figure out what that circuit does.[xxiii] We do know that the neocortex is constantly making predictions.[xxiv] These are the models we form of the world. So how do the networks of neurons in the neocortex learn predictive models? For example, when we touch a coffee cup but are not looking at it, if we move our finger, can we predict what we feel? Yes, we can. The cortex has to know that this is a cup, and where on the cup my finger is going to touch and how it’s going to feel. Our neocortex is making predictions about the cup.[xxv] By touching the cup in different areas, you can infer what the cup looks like, it’s shape, density and volume.[xxvi] If you touch the cup with three fingers at a time, each finger has partial knowledge of the cup and can make inferences and predictions about the whole cup as well.[xxvii] If you do this over a few objects, you get to know the objects and their features. Next time you touch an object that you have touched before, you can pretty quickly determine what you are touching and information you have about this.[xxviii] AI in this way would work as a neocortex and make predictions about something based on something like touch. A biological approach, also based on the workings of the neocortex, called the Thousand Brains Theory of Intelligence, says that our brain builds predictive models of the world through its experiences.[xxix] The Numenta team has discovered that the brain uses map like structures to build hundreds of thousands of models of everything we know. This discovery allows Jeff and his team to answer important questions about intelligence, how we perceive the world, why we have a sense of self, and the origin of high-level thought. This all happens in the neocortex which processes the changing time patterns and learns models of the world that are stored in memory and understands positioning. The Thousand Brains Theory says that these aspects of intelligence occur instantaneously – in no order.[xxx]
It is clear from many recent neuroscience scholarly articles and other ones such as Is the Brain More Powerful Than We Thought? Here Comes the Science that much inspiration and ideas still awaits us to help improve the state-of-the-art in machine intelligence.[xxxi] A team from UCLA recently discovered a hidden layer of neural communication buried within the dendrites where rather than acting as passive conductors of neuronal signals, as previously thought, the scientists discovered that dendrites actively generate their own spikes—five times larger and more frequently than the classic spikes stemming from neuronal bodies (soma).[xxxii] This suggests that learning may be happening at the level of dendrites rather than neurons, using fundamentally different rules than previously thought. This hybrid digital-analog, dendrite-soma, duo-processor parallel computing is highly intriguing and can lead to a whole new field of cognitive computing. These findings could galvanize AI as well as the engineering new kinds of neuron-like computer chips. In parallel to this, the article called “We Just Created an Artificial Synapse That Can Learn Autonomously” mentions a team of researchers from National Center for Scientific Research (CNRS) that has developed an artificial synapse called a memristor directly on a chip that are capable of learning autonomously and can improve how fast artificial neural networks learn.[xxxiii]
Although we are making progress on several fronts to get a better understanding of how the human brain functions, the simple truth is that there is still much to uncover. The more we do understand, the more we can apply this to understanding the machines we are creating. On the other side, the more we understand about how to develop intelligent systems, the more tools and insights are provided to enhance our understanding of how the brain works. This is truly exciting to both neuroscience and AI. And as we dive deeper into understanding how both work, and what it is that makes us human, we can also start thinking about a future that is truly human and empowered and aided by machines. Machine intelligence and human intelligence are interlinked. Because AI is reliant on humans, as humans we have the power to steer AI. To decide where it goes. To shape our lives, our world, and our future with the amazing tools at our disposal. This is not a job for the future. It creates the future. It is not a job for other people, it is up to all of us to decide what kind of world we want to live in…
(For more on this, read the paperback or e-book, or listen to the audiobook or podcast – see jacquesludik.com)
34. Lessons Learnt, Limitations, and Current State-of-the Art in AI
Rich Sutton, a Professor of Computer Science at University of Alberta, and Research Scientist at DeepMind, has shared some reflective thoughts on the last 70 years of AI research in his blog post The Bitter Lesson.[i] According to him the bitter lesson seems to be that general machine learning methods which leverage computation turned out to be significantly more effective than those that explored other methods such as leveraging human domain knowledge. It is clear that the exponentially declining cost per unit of computation that continued over decades as described by Moore’s law was a key contributor. Rich references how this has been clearly illustrated for computer board games such as chess and Go using search and learning-based approaches, speech recognition using deep learning and earlier on statistical hidden Markov models, and computer vision using deep learning convolution based neural networks. The bitter lesson is based on observations that followed a human-centric pattern where AI researchers started to build knowledge into their AI systems which did well in the short term and gave satisfaction to the researchers involved, but in the medium and longer term delivered no further improvements and even impeded further progress before being overtaken by competing approaches that scales computation by search and learning. The specific recommendation is that AI researchers and practitioners should not build in any part of arbitrary inherently complex systems that are being modeled as their complexity is typically unlimited; instead, they should focus on building in the meta-methods such as search and learning to detect and capture this arbitrary complexity. Therefore, the AI systems should search for good approximations and learn how to discover instead of being fed with content that we have discovered which makes it potentially more difficult to learn the discovery process.[ii]
We know that deep supervised learning works well for perception when there is abundant labeled data and deep reinforcement learning works well for action generation when trials are cheap such as within a simulation. We also know that deep learning has serious limitations. In Francois Chollet’s blog on The limitation of Deep Learning he summarizes the true success of deep learning thus far as the ability to map an input space to a target space using a smooth and continuous geometric transform that typically consist of multiple connected layers that forms a sufficiently large parametric model and trained by sufficiently huge amounts of labeled and annotated data. The weights of the layers are the parameters of the differentiable geometric transform which are updated in an iterative fashion by a gradient descent algorithm based on the model’s performance. The spaces in the model have sufficiently high dimensionality to capture the full extent of the relationships found in the original input data. Although the number of possible applications and the application potential of deep learning models are vast, it can only solve problems that can be addressed with its sequence of continuous geometric transformations that maps one vector space to another. A key limitation of deep learning is that even with unlimited data available or adding more layers, it cannot handle algorithmic type of data manipulation, reasoning, or long-term planning. Most software programs cannot be expressed as deep learning models due to not having sufficient data available to train it, or not being learnable in the sense that the geometric transform is too complex or there does not practically exist a deep learning model that corresponds to the program as only a small subset of all possible programs can be learned using deep learning. There is also a risk with anthropomorphizing machine learning models as can be seen with the classification of contents in pictures along with the generation of captions associated with pictures that give people the false impression that these models understand the contents in the pictures. This is illustrated with special adversarial input examples that slightly modify an image by adding a class gradient to deceive the model in predicting an incorrect class. For example, by adding a “gibbon” gradient to a picture of a panda, the model classifies the panda image as a gibbon. This not only demonstrates how fragile these machine learning models can be, but also that it understands and interprets their inputs in a way that is not relatable to the embodied way that humans understand sensorimotor inputs and experience. Francois also argues that there is a fundamental difference in the nature of the representations formed by deep learning models and human brains, where the generalization performed by the these deep learning models are more local and requires lots of training examples compared to extreme generalization formed by the abstract models in the human mind that are able to perform abstraction, reasoning, long term planning and adaptation to new or imagined experiences, situations, concepts, objects, or information that could be substantially different to what was observed before with a lot less examples. In comparison, the more local generalization power of deep learning networks within the context of pattern recognition is shown in how it adapts to novel data that is much closer to the historical data it was trained on. Francois suggests that a potential substrate for abstract modeling and reasoning could be computer programs, which will be discussed further later in this chapter.[iii]
Like many other AI practitioners and researchers also recognize, Andrew Ng also sums up the limitations of deep learning to be the inability to handle causality, adversarial attacks, explainability, and learning from small data sets. Yan LeCun agrees with this assessment and highlights the challenges with deep learning as learning with fewer labeled samples and/or fewer trials where self-supervised and unsupervised learning can be used for learning to represent the world before learning tasks, learning to reason by making it more compatible with gradient-based learning, and learning to plan complex action sequences by learning hierarchical representations of action plans.[iv] Judea Pearl calls the deep learning style of machine learning a form of “model-blind curve fitting” that is unable to address “what if” type of interventional, counterfactual or retrospective questions, and not able to do causal reasoning or being transparent in terms of explainability or interpretation.[v] It is also clear that there is much room for improvement with respect to transfer and multi-task learning and making better use of unlabeled data. Gary Marcus and Ernest Davis in Rebooting AI: Building Artificial Intelligence We Can Trust identifies three core problems with deep learning type of machine learning which includes that it is greedy with respect to the training data required (e.g. AlphaGo required 30 million games to reach superhuman performance), its opaque in terms of not being easy to understand why it makes mistakes or why it fairs very well, and its brittleness as shown above with the miss classification of slightly modified images.[vi] In Deep Learning: A Critical Appraisal Gary expands on this and highlights ten challenges that faces the current deep learning systems: Apart from being fragile in certain instances as well as data hungry, deep learning has limited capacity for transfer learning, mainly extracts superficial patterns, have no natural way of dealing with hierarchical structure where larger structures can recursively be constructed from smaller components, is struggling with open-ended inference, is not sufficiently transparent, has so far not been well integrated with prior knowledge, it cannot inherently distinguish between causation and correlation, it assumes a predominantly stable world and struggles to deal with systems that are continuously changing with varying rules, its predictions and classifications cannot be always trusted, and in general not easy to engineer with from robustness and even replicability perspective.[vii]
(For more on this, read the paperback or e-book, or listen to the audiobook or podcast – see jacquesludik.com)
35. Progress, Priorities and Likely Future Paths for AI
As we consider the progress, priorities, and likely future paths of AI, this section is anchored by exploring a better way of measuring intelligence for AI systems as this would be one sensible way of getting relevant feedback on the true progress that we are making in developing better AI systems and also possibly help to direct and prioritize future AI research and engineering paths. Earlier in this chapter, I have referenced Piero Scaruffi’s complaint about no significant progress being made in the development of abstract algorithms that improve automatic learning techniques. He describes today’s deep learning as a smart way of number crunching and manipulating huge data sets in order to classify or predict data which is driven by increased computing power and lots of data without any groundbreaking paradigm shift.[i] In François Chollet’s paper On the Measure of Intelligence he draws special attention to the many defects of the current AI research agenda and reasons for a psychometric and ability-based assessment of AI systems, which allows for a more well-grounded, fair and standardized comparison between not only human and machine intelligence, but any two intelligent systems.[ii] As mentioned earlier in this section there are at least two divergent visions for intelligence where the one sees it as the ability to execute a collection of task-specific skills and the other as a generalized learning and skills-acquisition capability. One of the current shortcomings is a focus on benchmarking intelligence by comparing the skill exhibited by AI systems and humans at specific tasks such as video and board games. This approach is limiting and not a true reflection of intelligence as it is not measuring the skills acquisition capability that also takes prior knowledge and experience in consideration to showcase the true generalization capability with respect to robustness, flexibility, and generality along with the adaptability of the intelligent system. The performance evaluation of skills-based narrow AI systems is typically done via a human review, a whitebox analysis that inspects the implementation of the system, benchmarking against a known test set of inputs, or competition against humans or other AI systems. When considering evaluating generalization capability it makes sense to categorize the degrees of generalization which can include zero generalization capability with systems that are applied to tasks or applications with no novelty or uncertainty, local generalization or robustness that is displayed in systems that can for example handle new points from a known distribution, broad generalization or flexibility where systems handles a broad range of tasks or applications without human intervention, or extreme generalization that is demonstrated in open-ended systems. He also differentiates between system-centric and developer-aware generalization where the latter refers to the ability of an AI system that handles situations that both the system as well as the developer of the AI system have not seen before whereas in the former case it is just the system that has not encountered the new situation. Francois Chollet argues against a more universal generalization (also called universal g-factor) from a “no free lunch theorem of intelligence perspective” as all known intelligent systems such as humans are typically conditioned on their environment and optimized to solve their own specific problems. So universal intelligence according to him does not provide any “shortcuts” in this regard as any two AI systems which include human intelligence are equivalent when their performance is averaged across every possible problem from a uniform distribution over the problem space. So, all our definitions of intelligence are for all practical purposes only relevant within a human frame of reference. This goes directly opposite the viewpoints from Universal Psychometrics or Universal Intelligence (by Shane Legg and Marcus Hutter) that completely rejects an anthropocentric approach and aims to use a single absolute scale to measure all intelligent systems.[iii]
(For more on this, read the paperback or e-book, or listen to the audiobook or podcast – see jacquesludik.com)
BicStreet
This Democratizing AI Newsletter coincides with the launch of BiCstreet‘s “AI World Series” Live event, which kicked off both virtually and in-person (limited) from 10 March 2022, where Democratizing AI to Benefit Everyone is discussed in more detail over a 10-week AI World Series programme. The event is an excellent opportunity for companies, startups, governments, organisations and white collar professionals all over the world, to understand why Artificial Intelligence is critical towards strategic growth for any department or genre. (To book your tickets to this global event click the link below and enter this Coupon Code to get 5% Discount: Enter Coupon Code: JACQUES001 (Purchase Tickets here: https://www.BiCflix.com; See the 10 Weekly Program here: https://www.BiCstreet.com)).
Background LinkedIn Articles on Democratizing Artificial Intelligence
AI as Key Exponential Technology in the Smart Technology Era
AI-driven Digital Transformation of the Business Enterprise
AI Revolutionizing Personalized Engagement for Consumer Facing Businesses
AI-powered Process and Equipment Enhancement across the Industrial World
Ultra-personalized AI-enabled Education, Precision Healthcare, and Wellness
AI’s Impact on Society, Governments, and the Public Sector
Audiobook “Democratizing AI to Benefit Everyone” available world-wide
Launching the Jacques Ludik Podcast and YouTube Channels – Democratizing AI To Benefit Everyone
The audio book version of “Democratizing Artificial Intelligence to Benefit Everyone” is also available via major audio book market place world-wide. See details on my website as well as below. You can also listen to audio content of this book on the Jacques Ludik YouTube Channel or Jacques Ludik Podcasts. This release is in follow-up to the e-book (Kindle) and paperback version of the book that was released earlier this year on Amazon with some further updates recently.
For some background, see also the following introductory articles Democratizing AI to Benefit Everyone and AI Perspectives, Democratizing Human-centric AI in Africa, and Acknowledgements – Democratizing AI to Benefit Everyone (as well as United Nations & Democratizing AI to Benefit Everyone; World Economic Forum and Democratizing AI to Benefit Everyone; OECD and Democratizing AI to Benefit Everyone; AI for Good and Democratizing AI to Benefit Everyone).
For further details, see jacquesludik.com.
References
Making Sense of the AI Debates
[ii] Nick Bostrom, Superintelligence: Paths, Dangers, Strategies.
[iii] Max Tegmark, Life 3.0.
[iv] https://futureoflife.org/background/benefits-risks-of-artificial-intelligence/?cn-reloaded=1
[v] Max Tegmark, Life 3.0.
[vi] https://www.theregister.com/2015/03/19/andrew_ng_baidu_ai/
[vii] Stuart Russell, Human Compatible: Artificial Intelligence and the Problem of Control.
[viii] https://www.nytimes.com/2017/09/01/opinion/artificial-intelligence-regulations-rules.html?ref=opinion
[ix] Hans Moravec, Mind Children: The Future of Robot and Human Intelligence; Ray Kurzweil, The Age of Spiritual Machines; Ray Kurzweil, The Singularity is Near: When Humans Transcend Biology.
[x] Ray Kurzweil, The Singularity is Near: When Humans Transcend Biology.
[xi] https://idlewords.com/talks/superintelligence.htm
[xii] https://www.theatlantic.com/science/archive/2018/11/diminishing-returns-science/575665/
[xiii] https://fchollet.com/blog/the-singularity-is-not-coming.html
[xiv] https://medium.com/@francois.chollet/the-impossibility-of-intelligence-explosion-5be4a9eda6ec
[xv] https://rodneybrooks.com/the-seven-deadly-sins-of-predicting-the-future-of-ai/
[xvi] https://rodneybrooks.com/the-seven-deadly-sins-of-predicting-the-future-of-ai/
[xvii] https://arstechnica.com/cars/2020/08/teslas-slow-self-driving-progress-continues-with-green-light-warning
[xviii] https://www.theringer.com/tech/2019/5/16/18625127/driverless-cars-mirage-uber-lyft-tesla-timeline-profitability;
[xix] https://www.scaruffi.com/singular/download.pdf
[xx] https://www.scaruffi.com/singular/download.pdf
[xxi] https://futureoflife.org/superintelligence-survey/?cn-reloaded=1; https://bigthink.com/surprising-science/computers-smart-as-humans-5-years?rebelltitem=1#rebelltitem1
[xxii] https://bigthink.com/surprising-science/computers-smart-as-humans-5-years?rebelltitem=1#rebelltitem1
[xxiii] https://youtu.be/Me96OWd44q0
[xxiv] https://youtu.be/_L3gNaAVjQ4
[xxv] https://spectrum.ieee.org/computing/software/humanlevel-ai-is-right-around-the-corner-or-hundreds-of-years-away
[xxvi] https://aiimpacts.org/ai-timeline-surveys/
[xxvii] https://rodneybrooks.com/agi-has-been-delayed/
Human Intelligence versus Machine Intelligence
[i] https://en.wikipedia.org/wiki/Intelligence
[ii] Shane Legg and Marcus Hutter. A collection of definitions of intelligence, 2007.
[iii] https://arxiv.org/pdf/1911.01547.pdf
[iv] Max Tegmark, Life 3.0; https://youtu.be/e3K5UxWRRuY
[v] https://youtu.be/e3K5UxWRRuY
[vi] https://youtu.be/e3K5UxWRRuY
[vii] https://www.youtube.com/watch?v=xqetKitv1Ko
[viii] https://www.youtube.com/watch?v=xqetKitv1Ko
[ix] https://www.youtube.com/watch?v=xqetKitv1Ko
[x] Frank Wilczek, The Unity of Intelligence, an essay in John Brockman, Possible Minds: Twenty-five Ways of Looking at AI.
[xi] Frank Wilczek, The Unity of Intelligence, an essay in John Brockman, Possible Minds: Twenty-five Ways of Looking at AI
[xii] Frank Wilczek, The Unity of Intelligence, an essay in John Brockman, Possible Minds: Twenty-five Ways of Looking at AI
[xiii] Frank Wilczek, The Unity of Intelligence, an essay in John Brockman, Possible Minds: Twenty-five Ways of Looking at AI
[xiv] Yuval Noah Harari, Homo Deus.
[xv] https://www.youtube.com/watch?v=-EVqrDlAqYo&feature=youtu.be
[xvi] Lisa Feldman Barret, How Emotions Are Made; Lisa Feldman Barret; Lisa Feldman Barret, Seven and a Half Lessons About the Brain.
[xvii] Lisa Feldman Barret, How Emotions Are Made.
[xviii] Lisa Feldman Barret, Seven and a Half Lessons About the Brain.
[xix] https://youtu.be/OheY9DIUie4
[xx] https://youtu.be/OheY9DIUie4
[xxi] https://www.youtube.com/watch?v=-EVqrDlAqYo&feature=youtu.be
[xxiii] https://www.youtube.com/watch?v=-EVqrDlAqYo&feature=youtu.be
[xxiv] https://www.youtube.com/watch?v=uOA392B82qs
[xxv] https://www.youtube.com/watch?v=uOA392B82qs
[xxvi] https://www.youtube.com/watch?v=uOA392B82qs
[xxvii] https://www.youtube.com/watch?v=uOA392B82qs
[xxviii] https://www.youtube.com/watch?v=uOA392B82qs
[xxix] https://www.youtube.com/watch?reload=9&v=6ufPpZDmPKA
[xxx] https://www.youtube.com/watch?v=-EVqrDlAqYo&feature=youtu.be
[xxxi] https://singularityhub-com.cdn.ampproject.org/c/s/singularityhub.com/2017/03/22/is-the-brain-more-powerful-than-we-thought-here-comes-the-science/amp/
[xxxii] http://www.physics.ucla.edu/~mayank/http://www.physics.ucla.edu/~mayank/
[xxxiii] https://futurism.com/we-just-created-an-artificial-synapse-that-can-learn-autonomously/; http://www.cnrs.fr/index.php; http://www2.cnrs.fr/en/2903.htm
Lessons Learnt, Limitations, and Current State-of-the Art in AI
[i] http://www.incompleteideas.net/IncIdeas/BitterLesson.html
[ii] http://www.incompleteideas.net/IncIdeas/BitterLesson.html
[iii] https://blog.keras.io/the-limitations-of-deep-learning.html
[iv] Yann LeCun, Self-supervised Learning, https://drive.google.com/file/d/1r-mDL4IX_hzZLDBKp8_e8VZqD7fOzBkF/view
[v] Judea Pearl, The Limitations of Opaque Learning Machines, an essay in John Brockman, Possible Minds: Twenty-five Ways of Looking at AI.
[vi] Gary Marcus and Ernest Davis, Rebooting AI, Building Artificial Intelligence We Can Trust.
[vii] https://arxiv.org/ftp/arxiv/papers/1801/1801.00631.pdf
Progress, Priorities and Likely Future Paths for AI
[i] https://www.scaruffi.com/singular/download.pdf
[ii] François Chollet, On the Measure of Intelligence, https://arxiv.org/abs/1911.01547.
[iii] Shane Legg, Marcus Hutter, Universal Intelligence: A Definition of Machine Intelligence, https://arxiv.org/abs/0712.3329.