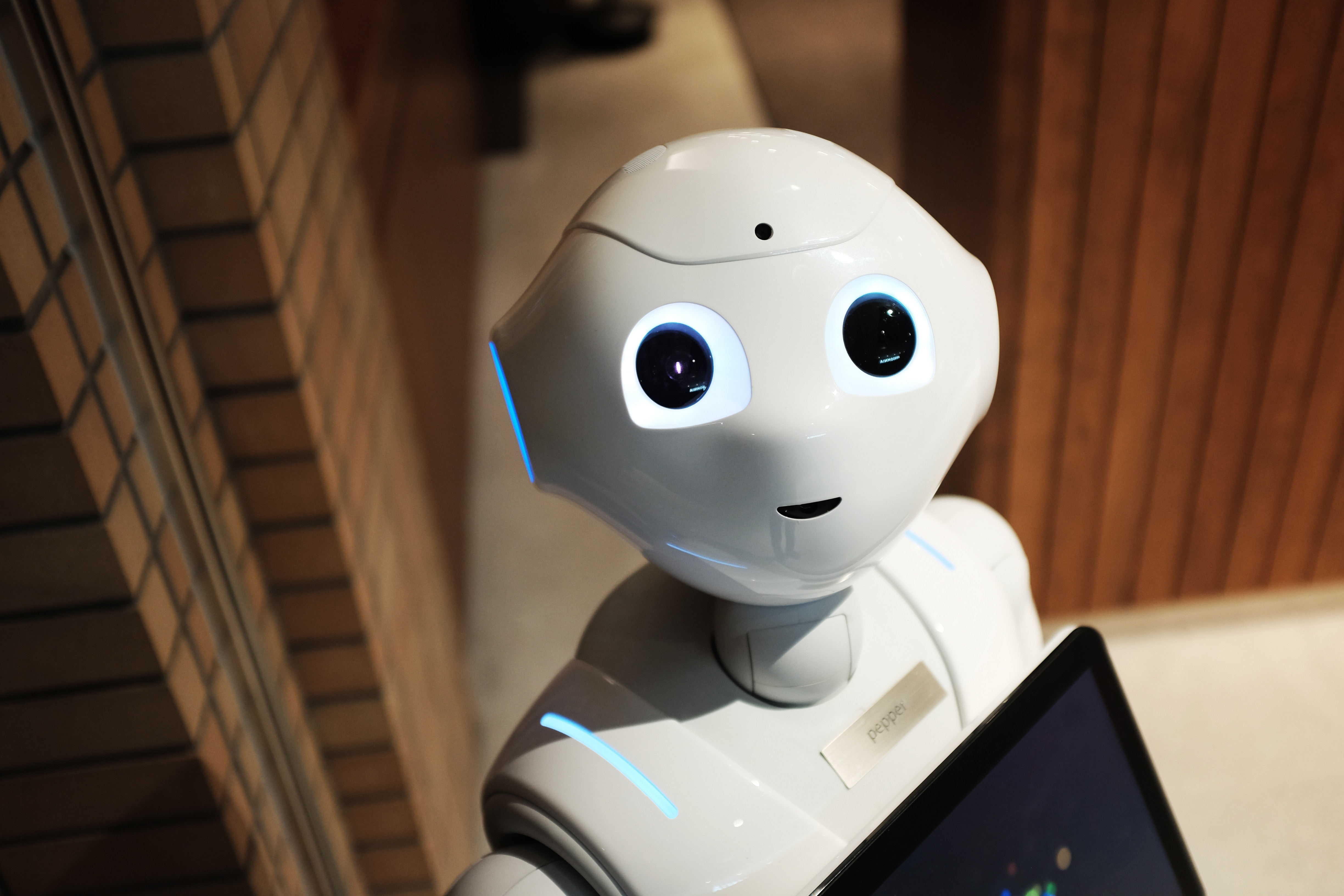
6 Factors to Consider as an AI Start-Up
With the aid of Smart Technology, record-breaking numbers of people are finding gaps in the market, better ways to do things, pains to eradicate, as well as market-disrupting products and processes. Yet, so many of these remain ideas. So, we asked the following questions: How can entrepreneurs, in the 4th Industrial Revolution, turn their ideas into goals, and their goals into business? What are some of the factors that they need to consider?
1 | Global Policies & Standards
The Organisation for Economic Cooperation and Development (OECD), European Union (EU), World Economic Forum (WEF), and the International Organization for Standardization (ISO) are all concerned with policies and standards surrounding ethical practice and data protection. GDPR and POPIA, for example, are but the first of many related laws actioned to govern business practices. The EU launched the Ethics Guidelines for Trustworthy AI in 2018 and highlights the three components of trustworthy AI: It should be lawful, ethical and robust. All fundamental parts of any company’s strategic decision-making process, whether you’re a start-up or an established business. Make sure you’re clued-up on all the applicable laws and regulations – for your business and product development processes.
2 | The Data Play
Artificial Intelligence relies on data – without it, it’s rather “unintelligent” or “dumb”. It requires data to learn, compare, predict and analyse. If your data is unreliable, incomplete or biased, your deployment of AI in your own business, and in your customers’ business, might be untrustworthy, irrelevant, inviable or ineffectual. As a business owner, you need to consider ways of sourcing, storing, checking and analyzing data. You’ll also have to evaluate whether you have enough relevant data that’s integratable, comparable, manageable and securable.
3 | Open Source AI Technologies
Whether Machine Learning, Deep Learning or Neural Networks – there are several Open Source Technologies to power your AI start-up:
- PyTorch: An open source Machine Learning library used for applications such as computer vision and natural language processing.
- Theano: Seen as the foundation for AI technologies, with a library that allows you to simply define, optimise and assess mathematical expressions.
- Darknet: Predominantly known for its vast abilities in accurate image classification.
- Caffe’s: Most common uses are in research and large-scale industrial applications in speech and vision.
- TensorFlow: One of the most common Machine Learning technologies, perfect for research and training neural networks.
- MS Cognitive Toolkit: Focuses on Deep Learning training, allowing it to function like the human brain.
- Accord.NET: Excellent for scientific computation in data and image processing.
- DeepDetect: Provides its own API and is powerful analysing text / numerics and detecting objects.
- Scikit-Learn: Perfect for classification, regression and clustering.
These technologies are built in a variety of coding languages. Depending on your language preference, data and business outcomes, there’s certainly an Open Source technology suited to your needs.
4 | API Integration
The ability to integrate into other systems, even those that seem unrelated to your product, is the difference between sustainability and stagnation. By not allowing for API integration, your product’s use and reach is limited (at best), and could become redundant (at worst). To scale your business, enable your creation to be used in as many ways and instances as possible. Think of it this way:
You have a product that tracks employees to find their motivational triggers. Through this, projects, communications and teams are tailored to employees based on how their individual narratives would best respond. A potential customer is sold on you idea. They tell you that they use such-and-such collaboration tools, intranets and so forth – ready for integration. You walk out the door without a deal.
5 | Continuous Improvement
We all have ideas of what we’d like to achieve – a vision and a mission. Rushing to get there might mean missing out on valuable learnings, feedback, new directions and essential tweaks. Seeking absolute perfection before launching is unnecessary. There’s truly no such thing as perfect. If your focus is on making small, evidence-based, and regular adjustments and enhancements, you’re setting yourself up for success. In the world of Machine Learning particularly, it takes time to build up data for accurate inferences. Taking your time equals more data, which equals more accuracy and improvement.
6 | Money, Money, Money
Funding entities face unique challenges with Smart Technology start-ups. AI is still met with fear, emerging businesses and processes, unpredictable adoption, changing demands, growing competition and digital mistrust – posing great risks and introducing skepticism in the tech start-up space. However, you’ve taken a risk by starting a business you believe in and if you’re willing to go the extra mile, there are Angel Investors and Venture Capital companies willing to take risks too. Others may tell you to pitch to just anyone, but we advise you to think carefully and do your homework. Conduct thorough research and approach investors known for their willingness to invest in high-risk ideas, and who focus on investing in the specific industry.
Finally. You’re here because you’ve got something to offer – you’re not just another AI company. Find your niche, build your product and put it out in the universe.